In the course of the 11 months that we’ve collected data on COVID-19 testing and outcomes, we’ve learned that an understanding of state reporting schedules and how they impact COVID-19 testing and outcome data is crucial for accurate data analysis. Many metrics can look drastically different from day to day, but many of these immediate changes result from day-of-week effects rather than true trends in the trajectory of the pandemic.
Not all states report all data every day
The main driving factor behind day-of-week effects is that not all states report data every day. Currently, eight states or territories (excluding American Samoa, Northern Mariana Islands, and US Virgin Islands, which report data very irregularly) don’t report any data on one or more days a week, and several more states only partially update their data on one or more days.
Most often, these days of limited reporting occur on the weekend, with five states not reporting data on Saturdays, and four states not reporting on Sunday. Two states—Idaho and Washington—regularly report their data after 7:30pm Eastern Time, when we publish our daily update, so we don’t catch their data until the next day. As a result, their weekend data is reflected on our data for Mondays, further stretching out the effects of the weekend.
Weekend effects
On weekends, when fewer states are reporting, COVID-19 data seems artificially low, and we see dramatic decreases in the number of cases, tests, and deaths reported. To many viewers of data, this can seem like a sign that trends are improving. However, in the short term, changes stemming from weekend reporting schedules mimic true trends in data, which is why it’s important to consider the day-of-week effects.
These weekend changes are present on Saturdays, but become most pronounced on Sundays, when case, death, and test numbers drop, due in large part to several states not reporting data.
The majority of states report data as of the previous day, or two days ago, so much of the data we report is actually not from the current day. This means that many of these weekend changes are offset about a day from what we normally think of as the weekend, explaining why case numbers dip most dramatically on Sundays and Mondays.
Additionally, our dataset is by date reported, rather than the day of onset. This is one of the reasons why our dataset and charts may not match up exactly with other sources. The majority of cases, tests, and deaths reported on a given day were discovered several days ago. Deaths can have an especially long lag from time of death to the date that death is reported on a state’s COVID-19 dataset. (Death reporting is further complicated by the fact there are two dates associated with a death: the date a death was reported, and the date the death occurred. We’ve written about why we believe the former metric is more useful for understanding how the pandemic is trending.)
Mondays and Tuesdays
At the beginning of the week, we typically see a smaller percentage of weekly cases reported. The causes of this are complex and hard to pin down, but here’s what we suspect: On the weekends, fewer testing sites are open, meaning that testing capacity is limited and fewer people are able to get tested. Many doctor’s offices are closed or have limited hours, so it’s harder to seek medical attention—which is sometimes the push that people need to get tested. Additionally, many county and state public health departments are operating in a scaled-down way, so it takes longer for data to be entered into state databases and to then appear on state pages and dashboards.
Because these changes involve multiple aspects of the data pipelines as well as changes in human behavior, even states that report data every day experience day-of-week effects.
One national trend we’ve noticed is that hospitalizations tend to flatline—or at least change less drastically—on Mondays. This can be encouraging if hospitalizations are otherwise rising, or disheartening if hospitalizations are dropping rapidly. But much of the time, these patterns are a reflection of the unique quirks of the data rather than a true change in the trends of patients currently hospitalized with COVID-19.
Even if states aren’t reporting data on a given day, people are still being tested, hospitalized, and dying. Those cases, tests, and outcomes need to be reported at some point, which is why we usually see a pattern of spikes in the data a day or two after a day of reduced reporting.
Wednesday to Friday
As we move into the middle of the week, we start to see larger figures, in large part a reflection of more complete reporting from states. On a national level, we see the largest case, test, and death figures reported on Thursdays and Fridays.
On a state level, it’s not uncommon to see a state go from reporting five deaths on Monday to 25 deaths on Thursday or Friday. This usually isn’t an indication of a sudden spike in the number of new deaths reported, but rather a result of their reporting schedule, and some degree of catch-up from the weekend.
These changes are why it’s so important to understand and think about state reporting schedules—absent that understanding, it can be easy to misinterpret the data.
Best practices for interpreting COVID-19 data, accounting for day-of-week fluctuations
Over the course of the pandemic—and in consultation with public health professionals—we’ve developed several strategies for interpreting fluctuating COVID-19 data in a responsible way. Our recommendations include:
Look at current hospitalizations. We’ve found that current hospitalizations tend to be more stable than other metrics on weekends and holidays. Because they’re not a cumulative metric, current hospitalizations are generally less sensitive to changes in reporting cadence than other metrics. However, hospitalizations aren’t entirely immune to day-of-week effects, so they should still be interpreted with caution. We encourage reading all recent data notes when looking at an individual state, and looking at the past few days of data when analyzing national numbers.
Use seven and 14-day averages. Because seven and 14-day averages show the general trends of data over a period of time, they are less affected by day-of-week effects. In fact, the complexities of state reporting schedules and day-of-week effects are the main reasons we advocate for the use of averages.
Look closely at timestamps. Timestamps (lastUpdateET in our API) are a valuable piece of metadata which provide insight into the freshness of data. It’s important to note that timestamps are reported differently by different states, some states report when data is as of, and some states report when data was last updated. Because of this, they are best interpreted as a general sense of when data is from, rather than an exact date and time.
Day-of-week effects have a significant impact on COVID-19 data. While we can’t erase them, we can learn to mitigate their effects on data analysis through careful consideration of their impact.
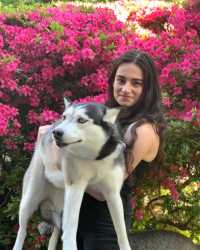
Hannah Hoffman is a data entry and data quality shift lead at The COVID Tracking Project and a student in the Washington, DC area.
More “Testing Data” posts
How Probable Cases Changed Through the COVID-19 Pandemic
When analyzing COVID-19 data, confirmed case counts are obvious to study. But don’t overlook probable cases—and the varying, evolving ways that states have defined them.
20,000 Hours of Data Entry: Why We Didn’t Automate Our Data Collection
Looking back on a year of collecting COVID-19 data, here’s a summary of the tools we automated to make our data entry smoother and why we ultimately relied on manual data collection.
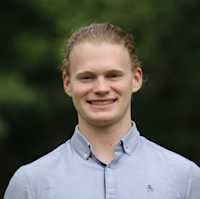
A Wrap-Up: The Five Major Metrics of COVID-19 Data
As The COVID Tracking Project comes to a close, here’s a summary of how states reported data on the five major COVID-19 metrics we tracked—tests, cases, deaths, hospitalizations, and recoveries—and how reporting complexities shaped the data.
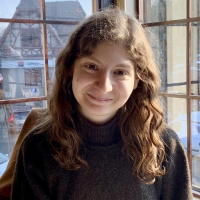
