Every day, our volunteers collect data on COVID-19 testing and outcomes from state health department websites. Although this may seem like a straightforward process, collecting all 820 of our data points consistently requires organization and planning. To make sure that we capture the same data point for each metric each day, we refer to a collection of “source notes”—a set of instructions for finding the data on state pages and dashboards. Today, we’re publicly releasing those notes.
We need such detailed source information because the 56 states and territories we track use many different terms and definitions for the same data points, and we want to make sure we interpret them correctly and assign them to the right categories. Our source notes are based on information available on public health department websites, our email and phone outreach to states and territories, and our ongoing internal analysis of the data.
Until today, we’ve made our sourcing traceable by posting the screenshots we capture of state health department websites each day: The screenshots currently cover 93 percent of our data points. We hope that releasing our internal source notes will make it even easier for members of the media and the public to locate exact data points on state websites.
What sources we track
Excluding state metadata (like state names, primary website, etc.) and calculated fields (like how much a metric has changed since the previous day), we capture 31 metrics in our API for our core testing and outcomes dataset. However, as you’ll have noticed if you’ve used our data, we don’t capture 31 data points for each state. Some metrics, like our main cases field, are reported by all 56 states and territories, while others, like tests pending, are reported by only four or five states or territories.
There are also several fields which track the same kind of metric—for example, total tests—in different units. Some states report these metrics in multiple units, and some report them in just one. These situations are where data sourcing plays a crucial role: It allows us to understand what a state’s number maps to in our API.
Data sources are constantly changing
Each day, states and territories add new metrics, change data definitions, and make clarifications on their COVID-19 sites and dashboards. For example, “Total PCR tests” might include only PCR tests on one day, and then include both PCR and antigen tests on the next—sometimes without so much as a footnote or a name change to mark the switch, only a big jump or dip in the numbers. Because we visit state websites to collect this data every day, we’re well positioned to spot these changes quickly.
Once we notice a change in a source during our daily publication shift, our data quality team examines the change and decides on the best course of action. This change can be as simple as tweaking the language in a source note, but it could also be complicated, requiring us to recategorize a metric, reach out to the state, or look for an alternate source of data.
States also continue to add new data points. To allow ourselves time to research a source, we rarely add data points the first day we see them. Instead, we note the existence of new data points and then analyze and evaluate them after our publication shift for addition to our tracking the next day. For both new data points and changes to existing ones, we’ve developed policies to allow us to do this source-evaluation work in a consistent way across data-entry shifts and across jurisdictions.
What do we look for in a source?
When a source changes—or appears for the first time—we consider three main factors in our decision to track it: accuracy, reliability, and usability.
Accuracy is the first and more important factor we look at—we want to see whether the numbers we’re looking at seem realistic based on other data we already have for a state. Although we only report data from official, public state government sites, and can’t control data abnormalities which arise from there, we also try to make sure that all the data we compile is intended for public consumption and has therefore undergone basic quality control from the state.
Reliability is the second factor we consider. Wherever possible, we choose metrics that are consistently updated, ideally every day. We encourage the use of 7 or 14-day averages when analyzing our data, but even those metrics can be affected by repeated days of missing updates. We also try to avoid data which comes from sources that frequently crash or go missing.
Reliability is relative, and varies depending on the kind of metric. For example, cases reported weekly would be an unreliable metric, since most states report that number daily. However, many states report recoveries weekly, and while it is certainly ideal to have them reported daily, we’d consider that a much more understandable situation than reporting cases weekly—especially given the amount of investigation required to report a recovered number based on symptomatic evidence, as some states do.
The last factor we look for in a source is usability. In large part because we think it’s so important to be aware of changes in sources and definitions, we manually collect our data rather than relying on scrapers, which means that a person enters every value.We want to make sure that we can realistically capture each data point—which, if the source is a summed graph that is not machine readable or legible to a human, we very occasionally can’t. To assist with capturing values that are hard to calculate manually, we use a notebook which runs complicated calculations for us. For numbers which are reported as daily rather than cumulative totals (which is how our API stores them), we sum them everyday to get a cumulative number. Additionally, to be usable, the data must be posted publicly and freely available; we don’t compile numbers privately given to us.
Takeaways for viewers of our source notes
Starting today, our source notes are publicly available. A few things to keep in mind when looking at them:
Our source notes spreadsheet is constantly being updated. It’s best to check the source notes regularly to ensure you’re getting the most up to date information
Data anomalies happen! These source notes explain where we get our data from, but don’t explain movements in the data itself. The public notes we add to our API and site pages for each state and territory usually include everything we’ve learned about anomalous reports.
Every source note is evaluated by our data quality team and then handwritten. If a source note does not quite match up with what you’re seeing on a state site, the site may have changed so recently that we haven’t yet updated the notes.
For more general information about our data entry and data quality processes, you can see our data policy overview, read our data definitions and FAQ, and look through our guidelines on using and visualizing the data in a responsible way.
Many people at The COVID Tracking Project are essential to creating and maintaining the source notes. Among them, we would like to thank Brandon Park for creating the syntax.
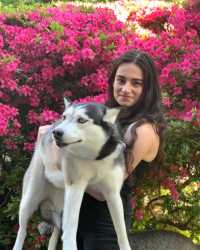
Hannah Hoffman is a data entry and data quality shift lead at The COVID Tracking Project and a student in the Washington, DC area.
More “Testing Data” posts
How Probable Cases Changed Through the COVID-19 Pandemic
When analyzing COVID-19 data, confirmed case counts are obvious to study. But don’t overlook probable cases—and the varying, evolving ways that states have defined them.
20,000 Hours of Data Entry: Why We Didn’t Automate Our Data Collection
Looking back on a year of collecting COVID-19 data, here’s a summary of the tools we automated to make our data entry smoother and why we ultimately relied on manual data collection.
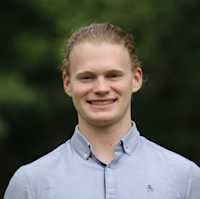
A Wrap-Up: The Five Major Metrics of COVID-19 Data
As The COVID Tracking Project comes to a close, here’s a summary of how states reported data on the five major COVID-19 metrics we tracked—tests, cases, deaths, hospitalizations, and recoveries—and how reporting complexities shaped the data.
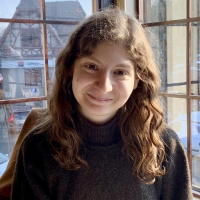
