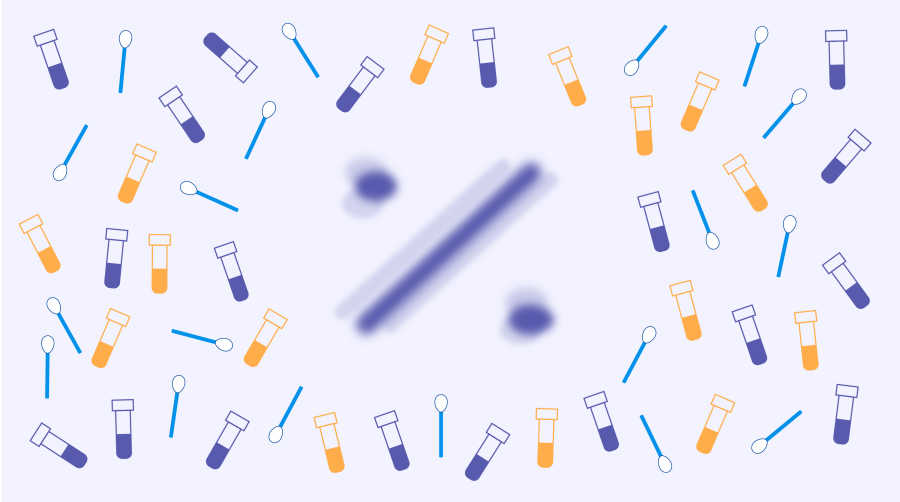
In almost every dashboard and plan for state and local reopenings—or re-closings—in the United States, the test positivity rate plays a starring role.
On the surface, the calculation for test positivity (also known as percent positive) is simple: Divide the number of positive tests by the total number of tests, in a select period of time, then multiply the result by 100. That number should give us the percentage of tests that have come back positive.
Test positivity can help us understand whether an area is doing enough tests to find its COVID-19 infections. The metric is widely used by local, state, and federal agencies to roughly gauge how well disease mitigation efforts are going. Put simply, when test positivity is high, it’s likely that not enough tests are being done and that most tests that are done are performed on symptomatic people. Both of these factors—insufficient testing and only testing people who feel sick—make it very likely that many cases are going undetected.
Unfortunately, there are two big problems with using test positivity as a sole or even most prominent way to measure an area’s performance and virus transmission. The first is that test positivity is not actually as easy to interpret as it looks: To understand what an area’s test positivity rate means, you need to know quite a bit more about that area’s viral prevalence (how many infections there are) and testing utilization (who is getting tested). This part is complicated enough that we wrote—and animated—a whole post about it. The very short version is that it’s impossible to interpret the metric accurately without looking at other factors, because test positivity reflects the percentage of people tested who have the virus, but not necessarily the percentage of the whole population that has the virus. But you should read the whole post; it’s great.
The second problem with relying on test positivity as a single measure of an area’s COVID-19 prevalence or response is that the underlying numbers used to calculate test positivity are counted differently in different places. In the absence of federal standards, US states and territories report their “total tests” data in several different ways. We also wrote a whole post about the many ways states report total tests and how we work with this data, if you want the deep dive.
Right now, our project compiles three ways of counting total tests: in “unique people” tested, in “test encounters” (unique people tested per day), and in “specimens tested.” Those different methods are best understood as producing three different testing metrics:
Unique people tested counts each person just once, no matter how many times they are tested over time, and no matter how many swabs are taken from them.
Specimens tested counts every sample taken from a person (via nose swab, for example) and run through a testing machine. Every time a person is retested, this metric counts them again. In some places and at some times, multiple specimens are taken from a single person—specimens tested counts each one separately.
Test encounters split the difference between the other two metrics. They count unique people tested per day. If a person is retested within a day, all the tests count as one test encounter. If they’re tested across multiple days, each new day counts as a new test encounter. If multiple specimens are taken for a single test, only the first one counts. Because retesting often takes place over many days, and we don’t generally see very many tests done using multiple specimens, test encounters usually produces a number slightly smaller than specimens tested, but often quite a bit larger than unique people tested.
The difference between these metrics is especially marked for states that do extensive retesting (or “serial” testing), since specimen and test encounter testing metrics capture retests, but the unique people testing metric does not.1
But why are we talking about these arcane differences in the way jurisdictions report tests? Because test positivity can change dramatically depending on which total test metric you use as the denominator. And different states, government agencies, and non-governmental institutions and projects prefer different testing metrics in their test positivity calculations.
Different denominators can produce wildly divergent test positivity rates
Consider the case of North Dakota. The state’s public health authorities calculate its test positivity rate as cases over tests, while until a few weeks ago, the Johns Hopkins Testing Tracker calculated North Dakota’s test positivity rate as cases over unique people tested.2 Because North Dakota does a very large number of retests, the state’s specimens-based total tests number was—and is—much higher than its unique people number. On September 10, 2020, the last day when Johns Hopkins and several other trackers used unique people tested as the denominator for the state's test positivity rate, North Dakota reported 333 new cases, 6,360 new total tests, and 1,333 new unique people tested, suggesting that North Dakota was doing a lot of retesting.
This difference in total test counts produces huge differences in test positivity calculations. On September 10, North Dakota’s test positivity rate was 5.2 percent if you calculated it using the larger (specimens) testing metric—and this is how the state calculated it for their official COVID-19 dashboard. But if you calculated it using the smaller (unique people) testing metric—as Johns Hopkins and several other third-party organizations did, using the data we compile—you would get a test positivity rate of 24.9 percent.
Importantly, neither of those test positivity rates is actually wrong—they just measure different things. But which way is the most useful right now? We took a look at the way various government agencies or public health organizations do the calculation.
Three main ways to calculate test positivity
The US Centers for Disease Control and Prevention offer a reasonably detailed discussion of the various ways to calculate test positivity, including a version of the following figure.
Importantly, each of these methods of calculating test positivity provides a different view of a given outbreak, and of an area’s response.
Method 1 (test over tests) uses a numerator and denominator that both include retests, which makes it helpful in understanding how many tests are being done compared to the number of infections in a given area.
Method 2 (people over tests) pairs a numerator that excludes retests (people) with a denominator that includes retests (tests). In many jurisdictions, this method is the only option based on the available data.
Method 3 (people over people) uses a numerator and denominator that both exclude retests, and is therefore especially helpful in validating case count growth (or declines) in a given area.
Importantly, these methods often produce highly divergent test positivity rates. Only five US states publish all four metrics (positive tests, people with positive tests, total number of tests, and total people tested) required to calculate test positivity using all three methods. But even with a sample of only five states, we can see how widely the three methods can differ in practice.
Test positivity rates using state data compiled by CTP for the week 9/25-10/1, calculated as (week of positive tests or people)/(week of total tests or people tested):
State | Method 1 (tests/tests) | Method 2 (people/tests) | Method 3 (people/people) |
---|---|---|---|
StateMaryland | Method 1 (tests/tests)2.81% | Method 2 (people/tests)2.25% | Method 3 (people/people)5.57% |
StateMassachusetts | Method 1 (tests/tests)0.89% | Method 2 (people/tests)0.75% | Method 3 (people/people)3.33% |
StateMissouri | Method 1 (tests/tests)10.25% | Method 2 (people/tests)4.65% | Method 3 (people/people)17.18% |
StateRhode Island | Method 1 (tests/tests)1.15% | Method 2 (people/tests)0.87% | Method 3 (people/people)3.79% |
StateWyoming | Method 1 (tests/tests)5.89% | Method 2 (people/tests)6.23% | Method 3 (people/people)15.38% |
Which way is the right way?
The CDC indicates that it calculates test positivity as positive tests over all tests (in our terminology, we understand “all tests” to be “specimens tested”—method 1, in the CDC’s terms) with the following rationale:
Data received at the federal level are de-identified and, therefore, are not able to be linked at the person level for de-duplication. This prevents CDC use of methods 2 (people over test) and 3 (people over people) in Figure 1 above.
This explains how the CDC itself calculates test positivity, but avoids making any recommendations about how states and territories should calculate their own. As far as we can tell by reverse-engineering some of the reports they continue to choose not to publish, but which were leaked, the White House Task Force also calculates test positivity using positive tests over all (specimens) tests, or method 1.
Resolve to Save Lives, a nonpartisan public health initiative headed by Tom Frieden, a former head of the CDC, recommends using people-based testing metrics: “If possible to report on unique individuals rather than tests, then this is preferred and should be explicitly stated.”
The method Resolve to Save Lives recommends—method 3—is one that the CDC and White House Task Force claim to be unable to use because they work with de-identified data, but is a method available to any states that can keep up with the ongoing work of de-duplication required to identify unique people tested.
The Johns Hopkins Testing Tracker data team has confirmed that they calculate test positivity using cases as the numerator and The COVID Tracking Project’s totalTestResults API field as the denominator. This field uses test encounters if they are available, then falls back to specimens tested if they are available, then unique people tested where no other test metric is available.3
For our part, The COVID Tracking Project does not directly calculate test positivity rates—and we will not do so until we are confident in our ability to communicate precisely about these complex issues in our annotations and visualizations. Nevertheless, our data is being used to perform the calculation, and we want to demonstrate the variety of ways in which test positivity gets calculated.
We can’t fix the mess, but we can highlight it
After many conversations with state officials, public health groups, and members of our advisory board, we made several changes over the summer to the way we display and distribute total tests data to help bring these complexities and inconsistencies into the light. (We detailed these changes in our total tests blog post and on our total tests documentation page.)
Several states now report total tests in test encounters, our preferred metric for the charts and visualizations we produce, and in our totalTestResults API field. This change had the knock-on result of bringing North Dakota’s test positivity rate at the Johns Hopkins Testing Trends dashboard much closer to the state’s internally calculated test positivity rate. But on the national level, the confusion remains. To consider just one example, if we drop one state down on the map from North Dakota, South Dakota has a 23.6 percent seven-day average test positivity on the Johns Hopkins Testing Trends dashboard (and COVID Exit Strategy) right now, but an 11.1 percent seven-day average test positivity rate on the state’s official dashboard. Although there are many other factors that can produce variance between a state’s internally calculated test positivity rate and those derived from public data, many can be largely or entirely explained by the use of different total test metrics.
Until every state reports their most basic COVID-19 data in the same way, direct test positivity comparisons across states remain an intractable problem. In the meantime, acknowledging that we are all trying to make the least-worst decision available at any given time, we offer a few words of advice for our API users who calculate test positivity—and for the agencies and organizations using those calculations to make policy:
Before you calculate test positivity using the data we compile, we recommend a careful reading of the data definitions for each API field and our total tests documentation to see all the available options for calculating test positivity. It is important to note that not all total test units have complete time series available.
However you choose to calculate test positivity, we recommend including clear, prominent notes or disclaimers about the inconsistencies inherent in this dataset and the resulting variations in the calculated test positivity rates.
Given that some state governments set policy based on internal and third-party test positivity calculations, we recommend that our API users report test positivity as a seven-day rolling average, rather than a single-day value, to avoid the jitters and spikes produced by backlogs or other temporary data-reporting anomalies. (Note: Prolonged and truncated time series can significantly skew the percentages.)
No single measure of the US public health response to COVID-19 should be considered in isolation, and this is especially true of test positivity. Unless we can help data users understand how test positivity is calculated for each jurisdiction, it’s impossible to responsibly compare test positivity across states and territories that report in so many different ways—only some of which we’ve been able to address in this post. In our upcoming third post on test positivity, we’ll be publishing a comprehensive comparison of yet more factors that can produce substantial divergence in calculations across jurisdictions.
In the meantime, we would encourage our data users—including those in state governments seeking to measure the outbreaks of neighboring states—to use all available data points in their evaluations of any given state or territory’s outbreaks or public health response. COVID-19 case counts, testing totals, hospitalizations, and death figures all help us understand a given jurisdiction’s experience of the pandemic, particularly when viewed alongside public data on local testing strategies. And we particularly and emphatically recommend against an over-reliance on test positivity calculations to justify changes in public health responses or policies.
Credits
Graphics: Júlia Ledur
Research: Kara Schechtman, Rebecca Glassman
1 Some jurisdictions appear to include repeat positives in their test encounters and specimens totals, and some do not; our research on this additional level of complexity is still underway, and we'll report back as soon as we know more.
2 The Johns Hopkins calculation used unique people tested as the denominator for North Dakota's calculation because they use our totalTestResults API field, and our team hadn’t yet received the historical testing data from North Dakota that would allow us to switch this field to use test encounters. We have since received this data.
3 “Available” in this case means that the state or territory publishes a metric with a definition that roughly matches one of the above test metrics and has a complete time series. You can read lots more about this in our total tests documentation.
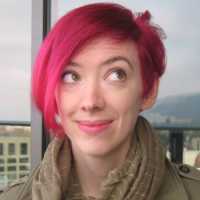
Erin Kissane is a co-founder of the COVID Tracking Project, and the project’s managing editor.
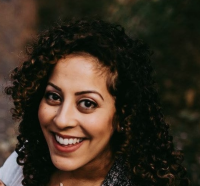
Jessica Malaty Rivera has an MS in Emerging Infectious Diseases and is the Science Communication Lead at The COVID Tracking Project.
More “Testing Data” posts
How Probable Cases Changed Through the COVID-19 Pandemic
When analyzing COVID-19 data, confirmed case counts are obvious to study. But don’t overlook probable cases—and the varying, evolving ways that states have defined them.
20,000 Hours of Data Entry: Why We Didn’t Automate Our Data Collection
Looking back on a year of collecting COVID-19 data, here’s a summary of the tools we automated to make our data entry smoother and why we ultimately relied on manual data collection.
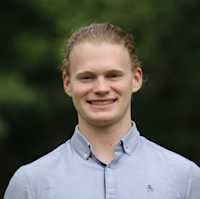
A Wrap-Up: The Five Major Metrics of COVID-19 Data
As The COVID Tracking Project comes to a close, here’s a summary of how states reported data on the five major COVID-19 metrics we tracked—tests, cases, deaths, hospitalizations, and recoveries—and how reporting complexities shaped the data.
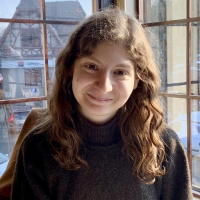
