Here at The COVID Tracking Project, we care most about making the data surrounding coronavirus testing transparent and publicly available. With this goal in mind, we hope to draw attention to a “new” metric that remains largely unexplored, but can help focus public attention on the need for more on testing. The question we should be asking ourselves is: Are we testing enough to get ahead of COVID-19? The tests-per-positive metric can help us answer it.
If you’ve been following COVID-19 data, you’ve probably encountered the “positivity rate” or “percent positive” metric. This tells us how many tests, out of all the tests conducted, have come back positive. When this percentage goes down, either an outbreak is fading or testing is rising—or both. For example, if a state’s positivity rate goes from ten percent (one in 10 tests comes back positive) to five percent (one in 20 tests comes back positive), that’s a major improvement, which could suggest that testing is now available for not just the most severe cases. This difference of five percent can have a tremendous epidemiological impact. While it does communicate an important piece of information, it’s not the most precise or immediately understandable metric.
However, if we flip the very same fraction upside down, no percentage calculations are necessary, and the math becomes much easier to grasp for the majority of people in the US, who may not have heard of the “positivity rate”. Instead of saying what percent of tests come back positive, we now focus on how many tests we have to perform to find a single new case. So instead of a “five percent positivity rate,” we would say that it takes 20 tests to find one positive, or 20 “tests per positive.”
This flipped-fraction is utilized by the gold standard for international testing statistics, OurWorldInData. Even though the two numbers are the same, their imagery tells a different story.
Visually, there are substantial advantages to using the tests-per-positive curve over the percent-positive curve. Unfortunately, the percent positive curve looks just like another curve that we need to flatten. Although the original “flatten the curve” figure was an effective public health visual, the over-reliance on the image and the oversimplified conversation has led to an undeniable amount of pandemic fatigue.
It’s also very easy to over-interpret a percent-positive graph as a “case curve,” when in fact percent positive tells you much more about how well (and who) you are testing than about viral prevalence. Tests per positive clearly focuses on testing and helps us avoid this misunderstanding. Using tests per positive also gives us a visual that highlights the work ahead of us. It’s important to clearly show the public what there is left to do, not just how bad we let things become. Using the tests-per-positive number can give us a new way to frame our response to the virus and help shift the narrative from pandemic progression to pandemic control.
It’s not just a question of a more optimistic view or replacing an exhausted flatten-the-curve visual, though. We know that the United States is aiming to improve its tests-per-positive metric daily. As the number of tests required to find a positive result gets larger, a percentage-based metric gets less useful. For example, 50 and 20 tests per positive is different by only three percent, visually. Three percent may seem small, but in this context, it is very meaningful. The percent-positive curve is poor at visually showing differences between these small percentages. Using tests per positive instead will emphasize them.
Catching up with more tests
This will also allow for a direct comparison of the United States towards other countries who were more successful in using testing to protect its citizens from the virus. Thailand and Australia are examples of nations that have regularly achieved more than 1,000 tests per positive. A curve depicting the equivalent percent-positive rate—0.1%—would be very difficult to read.
Tests vs. the virus
Many Americans are already asking how many tests are coming out positive. Using the tests-per-positive number gives us the chance to ask a more pointed, actionable question: “Are we testing enough to win the battle against the virus?” This question will be particularly important as more people, especially young people, return to work and social activities, and as clusters continue to spread nationwide. As exposure risk increases, so does the need for more testing. The more we test, the more cases we can identify—which is a good thing. And we can track how we are testing with tests per positive.
This measure of where we are on testing can help hold government officials and individual employers accountable for meeting this demand. Using tests per positive also helps journalists and science communicators continue to educate the public on important issues such as why we need 900K tests per day to safely reopen economies—and can help us avoid misunderstandings of the testing data that create a false sense of public security.
For data curators, news outlets, and government reporting agencies, the tests-per-positive curve tells a more compelling and accurate story than percent positive does. At its best, this graphic can redirect public skepticism about the severity of the virus to instead focus on an achievable goal: increasing testing in the United States.
Notes on making the switch
People who are already used to percent positive may find the tests-per-positive metric confusing— but if they already know the statistic, they are not the main audience that needs to hear this message. We have two small suggestions that, along with repetition and consistency, can help limit confusion.
If you use the tests-per-positive curve, label it with an upwards arrow that indicates "testing progress." Choose a cool color (not red). If no graphs are shown, use descriptive words like “progress” vs “setback” instead of nondescript arrows.
If you need to use the “positivity rate” graph for continuity, consider describing it using the fraction. If you write 6 percent, supplement or replace with “1 in 17 tests are positive” instead. Seventeen is the important number to see that is otherwise never calculated.
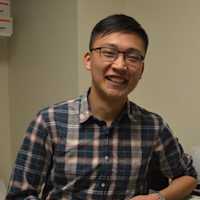
Tong Wang is an MD-PhD candidate at the University of Pennsylvania.
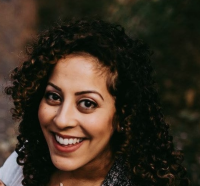
Jessica Malaty Rivera has an MS in Emerging Infectious Diseases and is the Science Communication Lead at The COVID Tracking Project.
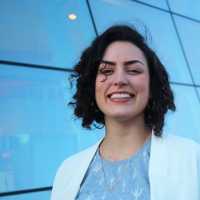
Júlia Ledur is a visual journalist and illustrator who leads the visualization team at The COVID Tracking Project. She is a former graphics reporter at Reuters.
More “Testing Data” posts
How Probable Cases Changed Through the COVID-19 Pandemic
When analyzing COVID-19 data, confirmed case counts are obvious to study. But don’t overlook probable cases—and the varying, evolving ways that states have defined them.
20,000 Hours of Data Entry: Why We Didn’t Automate Our Data Collection
Looking back on a year of collecting COVID-19 data, here’s a summary of the tools we automated to make our data entry smoother and why we ultimately relied on manual data collection.
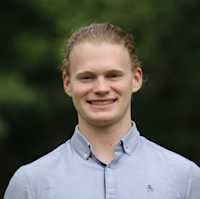
A Wrap-Up: The Five Major Metrics of COVID-19 Data
As The COVID Tracking Project comes to a close, here’s a summary of how states reported data on the five major COVID-19 metrics we tracked—tests, cases, deaths, hospitalizations, and recoveries—and how reporting complexities shaped the data.
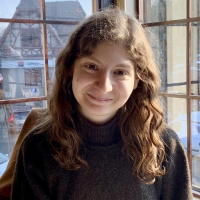
