If you’ve watched COVID-19 data over the past year, you are likely familiar with the phenomenon of a “death lag,” that a rise in cases does not immediately lead to a rise in deaths. This is for two reasons. The first is clinical: It takes time for COVID-19 to progress to the point of hospitalization or death. The second reason is an artifact of reporting. Deaths are complicated to record, and the infrastructure to record them is often outdated, so producing a comprehensive count takes time.
When the CDC released its COVID Data Tracker in May 2020, it followed in the footsteps of many other countries by establishing a “real-time” count it hoped would move faster than the traditional, sluggish method for counting deaths from a certain condition, which requires reviewing the text of death certificates. To assemble that real-time count, CDC turned to scraping data from state dashboards—much like external trackers such as our own.
This method did prove faster than more traditional methods for collecting deaths data. But that doesn’t mean it was fast enough. While the CDC has not shared much information on the average lag time for a death to register in state counts, we have long noticed distortions in state deaths data caused by lagging data followed by data dumps, from weekend dips to dramatic drops during holidays.1 As more data for the first year of the pandemic has become available, we can now put numbers to the reporting lags that shaped the CDC’s death count, as well as our own.
By comparing the CDC’s historical data to data reflecting the date those deaths actually occurred, we can see that there were reporting lags during all three surges of the US pandemic. As a result, the CDC’s data painted a misleading picture of COVID-19 mortality:
During the first and third surges, reporting delays led to delayed peaks in deaths in the CDC data.
During the second and third surges, the number of actual daily deaths exceeded the number of deaths that state health departments could count in a day. The ceiling on reporting led to persistent undercounts of deaths during peaks and dramatic data dumps after them.
These problems led to undercounts of daily deaths during surges and overcounts of daily deaths during declines. Because distortions tended to worsen around peaks, they may have obstructed our view of the pandemic at the moments that view mattered the most: when a better picture of COVID-19 mortality could have changed individual behavior or policy decisions.
How we measured the CDC’s data lags
We were able to quantify lags in the CDC’s dataset by plotting its historical data, which uses a date-of-report dating scheme, against historical data shared by some states that uses a date-of-death dating scheme.
Date-of-report data like the CDC’s does not represent deaths that actually happened on a given day, instead counting deaths that were reported by state health departments that day. This kind of data comes with the advantage that it can be reported in real time. However, it is muddled by reporting distortions: data dumps register as large spikes, and days affected by lags register as days with low deaths.
In date-of-death data, deaths are attributed to the dates they actually occurred, not the day they were reported. While counts for recent weeks can be incomplete and unreliable because of missing data, historical date-of-death data accurately reflects trends in COVID-19 deaths, as distortions like lags and data dumps are resolved by the backdating of deaths.
Now that the historical date-of-death data has solidified, charting the CDC’s date-of-report data against date-of-death data can reveal the extent of lags. It lets us see how many deaths actually occurred each day compared to how many were reported by CDC, or any other tracker that aggregated numbers from state dashboards.
Unfortunately, date-of-death data is hard to come by. The federal government does not provide it for data that is comparable to the counts in the COVID Data Tracker, so we have to source the data directly from states instead. Eighteen states, together accounting for about half of US deaths, provide this historical data on their state health department websites, enabling a comparison for a representative subset of states.2
Two kinds of death lags: slow counts and ceilings
Our comparison confirmed that there were substantial differences between CDC date-of-report and state date-of-death data that clearly indicated reporting lags.
In general, the CDC’s date-of-report data follows a simple pattern: the CDC’s count under-reported deaths during upswings, as states struggled to keep up during periods of rising volume. During downswings, the CDC data over-reported deaths as death counts caught up.
However, lagging death counts distorted trends differently for each surge. In the spring, lags result in the peak of deaths registering in the CDC’s data. In the summer, the actual peak of deaths corresponds exactly in the date-of-death and CDC data, but substantially undercount the actual daily deaths. Finally, in the winter, deaths in CDC data reached their peak about three days after the actual peak in deaths—but stayed there for nearly a month, as opposed to the actual deaths, which began to fall immediately.
These apparently idiosyncratic effects can be explained as a product of two different kinds of lags at play in the CDC’s deaths data, each of which shaped its counts to varying degrees over time. During the first surge, lags had a straightforward cause: processing speeds for deaths were slow. During the second surge, death counts lagged because the true number of daily deaths exceeded reporting capacity. And during the third surge, both kinds of lags came into play, causing especially dramatic distortions.
Slow reporting
The first kind of delay in death counts is simple: a lag between when a death occurs and when it shows up in a state’s death count because the process to count the deaths at the state level is slow. For example, if it usually takes a week for a state health department to learn about an individual’s COVID-19 death, then the trends in that state’s death data will also have a week’s delay.
The effect of this kind of lag was most pronounced in the first surge, when state public health departments were caught off-guard by the need to quickly tabulate counts of deaths caused by a certain condition—a process that is normally handled at the federal level. During the spring of 2020, the CDC’s death counts lagged by about eight days, as states struggled to figure out how to process the deaths in a timely way.
Over time, states improved their death reporting by taking measures such as speeding up the process for certifying deaths or upgrading their technical systems for handling death notification. These changes decreased the amount of time that it took for health departments to learn about a given death.
As a result, during the second surge, there was no reporting delay at all: deaths peaked in both the CDC’s data and the date-of-death data on August 5, 2020. In the third surge, it is a bit more complicated to assess the lag time, since deaths in the CDC data plateau at their highest point rather than peaking. But taking the midpoint of this plateau as the “peak” in CDC data, there appears to be a lag of about two weeks. It’s unclear what exactly caused slowness the second time around: possible factors are a rise in at-home deaths, which take longer to report, or the occurrence of further technical errors because of the strain.
Reporting ceilings
Just as states improved problems with reporting slowness, another problem opened up: states faced limitations on how many deaths they could process in a day in the first place. As deaths surged, daily counts surpassed that ceiling, resulting in persistent under-reporting of deaths and accumulation of a backlog for any deaths above the ceiling.
You might think that improvements to reporting speed would have also resulted in an increase of processing capacity by increasing throughput. Certainly, many of these improvements did increase capacity. But addressing slow reporting will not necessarily address one of the main causes of capacity problems, a reporting bottleneck. Along the chain of death reporting are many opportunities to speed up the process of death notification—for example, replacing faxed data with electronically transmitted data—that don’t save time of people like coroners, medical examiners, mortuary workers and clinicians who need to be involved in reporting every COVID-19 death. If those people are overextended, it doesn’t matter how fast the rest of your pipeline goes: You won’t be able to count any deaths beyond what those individuals can process.
States tried to address death reporting bottlenecks by expanding these workforces, but not enough to keep up with the spikes in deaths in later surges. And no amount of hiring could have accounted for the constraints imposed by holidays like Independence Day, Thanksgiving, and Christmas, all of which interrupted normal death reporting during surges. As a result, at the heights of the second and third surges, the CDC’s daily death tally didn’t bear much of a relation to how many deaths had actually occurred that day; instead, it represented how many deaths out of that total America could count.
There are two main signs that the CDC’s death count hit this limit. The first is, for both the second and third surges, the CDC data never reaches the same daily peak that registered in the date-of-report data: CDC data peaks 11% and 8% lower than the actual peaks, respectively. If the death counts during these surges had just been delayed, they would have eventually matched the heights of the date-of-death data.
The second is that, for both surges, deaths in the CDC data plateau at the very peak of the disease curve instead of reaching a clear apex. This pattern makes little sense when you compare the CDC data to what actually happened in the date-of-death data, which registers a clear peak followed by a decline. But accounting for the problem of reporting capacity, the period of time that deaths plateaued in CDC data represents the period when the actual death counts were above the reporting ceiling. Indeed, the length of time that deaths plateaued in CDC data during the third surge— 26 days—corresponds roughly to the length of time that deaths were above the value they plateaued at (1,667) in the date-of-death data, 33 days.
Reporting lags caused by hitting a ceiling are so insidious because they can cause distortions like the artificial plateaus. These are distortions that don’t just delay our understanding of trends but actually change the appearance of the trend.
The distortions extend beyond the peak of deaths to their decline. Any deaths that actually occurred above the reporting ceiling will eventually be reported in a backlog. Because these backlogs had longer to accumulate in the third surge, overcounting caused by data dumps increases between the second and third surge. (The decline after the third surge was also steeper than the second’s, making the differences especially dramatic).
Even before the date-of-death data solidified, we had some visibility into the extent of the backlogs from states that make data or notes about delayed processing of deaths available on their dashboard, such as Alabama, North Dakota and Michigan. And though it’s too early to do a full comparison of date-of-death data to date-of-report data from April 2021 onward, notes from states indicate they are still identifying deaths from the winter surge months after the peak, likely leading to continued overcounting of the death count in date-of-report data.
Why it matters
Any kind of lag in deaths is consequential because surging numbers of deaths, more than any other metric, tend to change people’s minds and behavior when we most need them to. Policymakers and institutions respond with regulations and precautions when they see an uptick in deaths. Researchers have even studied the possibility that individual changes in social distancing behavior motivated by rising death tolls can change epidemic curves. Because of comparisons to excess deaths, we can estimate that COVID-19 death metrics are large undercounts, which limited public awareness of the real toll from COVID-19. Reporting lags added another layer of undercounting to the death numbers during surges, which may have prevented curve-bending mitigation measures until it was too late.
Some amount of lag in deaths data is inevitable: Death is inherently complex, and it would be very hard to get the process of recording them to run in real time while also producing high-quality data. It would have been one kind of failure for US death data to exhibit undercounts just caused by reporting slowness in its COVID-19 death counts. But it is a failure of another level altogether that, at points of the pandemic, there were so many deaths that the United States’ public health infrastructure could not count them all. That kind of distortion keeps us from an understanding of the true shape of the pandemic until much later, when the date-of-death data is complete.
The simple fact that we could not count everyone who died of COVID-19 at the moments it was most critical sums up all that was wrong with the United States’ handling of the pandemic: the unquantifiable tragedy of the lost lives, and a public health data infrastructure too broken to collect the data that might have informed a response and prevented such loss.
Additional contributions from Alexis Madrigal, Joseph Bensimon, Michal Mart, Quang Nguyen, and Rebma.
1 We could not find any clear numbers from CDC on reporting lag time. The CDC provides median death reporting lags as part of its Pandemic Planning Scenarios modeling—broken down by age bracket—but the dataset they used to calculate the lags is unclear. Given that these numbers include an age breakdown, they likely used the line-level case surveillance dataset or NCHS death dataset instead of the aggregate dataset underlying the CDC Data Tracker, which doesn’t have any age data in it. The only time CDC has provided any information on lags in the aggregate dataset is in a June 2020 Morbidity and Mortality Weekly report, which included an upper-quartile lag on cases (15 days), but not deaths.
2 Data sources and underlying data used in this comparison can be downloaded here. The eighteen states represent 48% of US deaths as of May 13, 2021 according to the CDC’s death counts. To verify our findings on all states’ data, we also consulted the National Center for Health Statistics’ provisional COVID-19 death certificate data. NCHS data is organized by date of death, but it is not very comparable to CDC Data Tracker counts because the NCHS uses a different data collection pipeline and definition for deaths than most states. (By contrast, states tend to use the same definitions and processes to produce both the topline counts CDC scrapes and their date-of-death time series). Despite the differences between CDC Data Tracker and NCHS data, the comparison still affirms the overall trends.
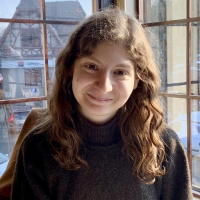
Kara Schechtman is Data Quality Co-Lead for The COVID Tracking Project.
More “Hospitalization and Death Data” posts
20,000 Hours of Data Entry: Why We Didn’t Automate Our Data Collection
Looking back on a year of collecting COVID-19 data, here’s a summary of the tools we automated to make our data entry smoother and why we ultimately relied on manual data collection.
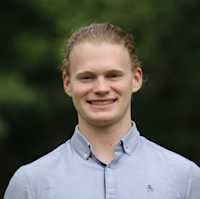
A Wrap-Up: The Five Major Metrics of COVID-19 Data
As The COVID Tracking Project comes to a close, here’s a summary of how states reported data on the five major COVID-19 metrics we tracked—tests, cases, deaths, hospitalizations, and recoveries—and how reporting complexities shaped the data.
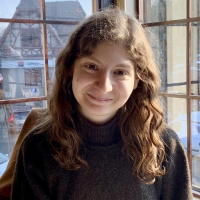

Releasing Our State COVID-19 Data Log
From July 2020 to March 2021, The COVID Tracking Project compiled a detailed set of structured COVID-19 data notes, both on changes states made to the data and changes we made to the data we captured from states. Today, we’re releasing those notes.
