On December 7, the US Department of Health and Human Services (HHS) published a facility-level COVID-19 hospitalization dataset dating back to July 31. This data provides a weekly view of how COVID-19 has hit hospitals across the country in more detail than ever before.
The HHS facility-level dataset includes metrics that allow us to look at individual hospitals with COVID-19 patients, determine how close they are to capacity, and track how their burden of patients has changed over time. More metrics may become available as the dataset is updated.
This dataset is a big deal—especially now, when hospitals are under unprecedented strain across the nation. This new HHS data, which includes counts of incoming COVID-19 patients, calculations on how many beds are available, and other hospital-related metrics by facility, allows us to see where COVID-19 is hitting healthcare systems the hardest. It’s also robust in ways that many other datasets aren’t; although counts of cases, tests, and deaths fluctuate around weekends and holidays, hospital workers never take a day off, and neither does their data.
As of December 10, The COVID Tracking Project counted over 107,000 Americans in the hospital with COVID-19, nearly 80 percent higher than at any previous time in the pandemic. This record level of hospitalizations is clearly alarming, but the strain on hospitals does not neatly map to state borders; hospital capacity is local, and state-level data can mask crucial problems in areas with few hospitals or surging outbreaks.
Because the dataset provides statistics at the facility level, we can also explore COVID-19’s effects on Hospital Service Areas (HSAs) and Hospital Referral Regions (HRRs). HSAs and HRRs are local and regional healthcare zones that are designed to more accurately show where Americans actually go for hospital care. As in a watershed, patients flow to certain spots for care, and these geographic areas don’t always align with individual counties or even state lines.
The numbers in this dataset come from hospitals themselves, which are required to report detailed capacity and utilization data on a daily basis. Hospitals primarily reported to the Centers for Disease Control and Prevention (CDC) until July, at which point responsibility for data collection switched to the HHS. Now, hospitals either report their data directly to HHS or report it to their state or state hospital association—agencies which are certified to report on the hospital’s behalf: The exact data reporting pipeline depends on the state.
Although the HHS faced challenges and questions over the summer surrounding their collection and reporting of hospitalization data, our researchers have watched the agency’s state-level dataset closely and now considers this data to be highly reliable and consistent with hospitalization reporting by the states themselves. We reported last week on why we believe HHS’s data is a useful complement to what states post.
What’s included in the data
This dataset provides a weekly view of each hospital facility’s status via the daily average or sum of metrics since the beginning of August. Here are the current categories of metrics (more detail is available in the associated data dictionary):
Facility information: hospital names, addresses, states, ZIP codes, hospital subtypes, FIPS codes (a unique identifier assigned to each US county), and CMS Certification Numbers (CCN).
COVID-19 patients in the hospital and in ICU: daily counts averaged over each reporting week, disaggregated by confirmed and suspected adult and pediatric patients in inpatient and ICU beds (except pediatric ICU hospitalizations).
Staffed bed capacity: daily counts averaged over each reporting week, broken down by various bed types: all beds (inpatient and outpatient), inpatient beds, inpatient adult beds, ICU beds, and adult ICU beds. These bed counts are inclusive of surge/overflow capacity.
New COVID-19 hospital admissions within each reporting week: weekly sum disaggregated by confirmed and suspected adult and pediatric patients as well as by age brackets (18-19, 10 year ranges from 20 to 79, 80+, and unknown).
Total ED visits associated with COVID-19 and overall: weekly sum of all emergency department (ED) visits regardless of reason and those visits defined as “related to COVID-19 (meets suspected or confirmed definition or presents for COVID diagnostic testing).”
Facility-level metadata: how many days the facility reported each required data element during a collection week.
Weekly confirmed influenza hospitalizations: currently optional, but some facilities choose to report this data.
We expect the dataset to be updated weekly on Mondays, with updates reflecting seven days of hospital reporting covering the prior Friday to Thursday. In the current dataset (released on December 7), the most recent week of data spans November 27 to December 3.
This dataset breaks out the numbers of patients in Short Term Acute, Long Term Acute, Critical Access, and Childrens’ Hospital facilities across the nation—which totals nearly 5,000 hospitals. As of the most recent reporting week, 95 percent of hospitals are reporting every required data element to HHS all seven days of the week.
It is also important to note that some types of hospitals are not fully represented in this dataset. Psychiatric and Rehabilitation hospitals are deemed lower-priority facilities in the COVID-19 response by HHS and not included here. US Department of Veterans’ Affairs, Department of Defense, and Indian Health Services facilities are also not included.
In addition, the hospitals reported in this dataset may not quite match up to the physical hospital campuses and names which you see driving around your area. When it is available, hospitals are reporting and identified based on codes called CCNs that are issued by the Centers for Medicare & Medicaid Services (CMS). One health system may report on behalf of multiple sites, or each hospital site within a system may report on its own, depending on how each system is set up for reporting to CMS. For example, in New York City, all NYU Langone hospitals share one CCN while three Mount Sinai hospitals have three different CCNs. In the occasional case that a hospital in the dataset does not have a CCN, it is given a non-CCN unique identifier code.
Data checks to keep in mind
Data journalists and health researchers have put together a community FAQ page which may answer many questions for those looking to use the HHS facility dataset themselves. Many thanks to collaborators at Careset Systems, the University of Minnesota, and COVID Exit Strategy for their work! We’ll focus on strategies for understanding and quality-checking the HHS numbers.
First, you can check how comprehensively the facilities in your area report to the HHS every week. The “coverage” numbers in the dataset for different metrics refer to the number of days that hospitals reported the corresponding metrics in a given week. A value of six for “inpatient_beds_used_7_day_coverage,” for example, indicates that for six out of seven days of that reporting week, this facility reported how many staffed inpatient beds are being used. While some facilities in earlier time points in the dataset have varied coverage, the vast majority currently report most metrics either six or seven days a week. HHS also provides a related public dataset and map showing latest reporting coverages per metric by facility—a reflection of HHS’s commitment to improving data collection and transparency.
Second, you can look at how often numbers from each facility are real outliers, such as reporting usage of beds over 100 percent capacity. The FAQ cited above provides sample formulas for calculating important ratios for a given hospital. Dividing “staffed_adult_icu_bed_occupancy_7_day_avg” by “total_staffed_adult_icu_beds_7_day_avg,” for example, should yield the share of adult ICU beds which are currently filled with patients.
If this figure is much over 100 percent, the hospital may have reported an erroneously high number for hospitalized patients or low number for beds. Occupancy percentages just above 100 percent may plausibly reflect temporary overflow of hospital bed capacity as hospitals lag slightly behind in staffing more beds or reporting these changes.
Since the data per facility covers every week since the beginning of August, we can also analyze occupancy and capacity changes of a specific hospital over time to assess whether high occupancy is a consistent and realistic pattern or an aberration of reporting.
For example, at Methodist Hospital in San Antonio, Texas, we see that the number and percentage of COVID patients occupying inpatient beds declines from around 200 (19 percent of capacity) in August to a low of 60 (6 percent of capacity) in October and then increases to 170 (9 percent of capacity).
However, at Houston Methodist West Hospital in Houston, Texas, we see an obvious outlier reporting nearly 600 percent occupancy of inpatient beds in the week of July 31. This is almost certainly an error and not reflective of the reality in that hospital. If we disregard that point, the pattern of hospitalizations and occupancy is similar to the San Antonio facility. The level of granularity of this dataset allows for specific analyses of hospitalization numbers at different stages of the pandemic, revealing insights that may be obscured in the national data.
For example, while only a few facilities were overcrowded in late August, many hospitals with an ICU were almost exclusively occupied by COVID-19 patients last week.
Spotting areas where COVID-19 has disproportionately affected ICU capacity across the country can help us identify regions that have been particularly challenged by the virus. While county- and ZIP-code-level data provide valuable insights, the HRR level may provide the most realistic picture of where patients are receiving care. These HRRs reflect how patients travel to their nearest or otherwise most accessible hospital, which may be located across county and state lines—such patterns are often obscured when analyzing along administrative borders.
We performed a preliminary investigation of the share of ICU beds that were occupied by COVID-19 patients. Our goal was to identify which HRRs had facilities that experienced the largest increase in COVID-19 ICU occupancy between the week of August 28 (the week at which we saw that hospital reporting errors began minimizing significantly) and the week of November 27 (the most recently available week of data).
Out of ten HHRs which saw the most drastic increase in COVID-19 patient occupancy, half are in the southwest United States. Three of these regions are in Texas bordering New Mexico: Amarillo (an increase of 70.9 percentage points), El Paso (an increase of 53.8 percentage points), and Lubbock (an increase of 47.7 percentage points). In contrast, only one HRR on the entire East Coast was represented in the top ten: Providence, Rhode Island, with an increase of 51.2 percentage points during this timeframe.
This type of analysis could be extended to look at other metrics or time periods within the dataset. It could also be applied to other geographic units like Hospital Service Areas (HSA) or Metropolitan/Micropolitan Statistical Areas (MSA), which may potentially yield varying results that are relevant at different spatial and administrative scales.
Future analyses
This new data released by the HHS represents the most spatially granular data ever collected about how COVID-19 is impacting hospitals. This new level of detail allows us to ask many questions that can provide specific insight on the impact of the pandemic on the US healthcare system, including:
What are the capacity needs for individualized health care systems to serve their COVID-19 and non-COVID-19 patients, and how has this evolved over time?
Are there regional differences in the size or shape of certain trends that we can observe by aggregating facility-level data at different geographic units (e.g., ZIP codes, counties, MSAs, HSAs)?
Have COVID-19 hospitalizations differentially impacted certain age groups (both pediatric and the 10-year bracketed adult age groups)?
The answers to questions like these can help decision-makers take clear and precise action. However, the depth and complexity inherent in these numbers require careful analysis to unlock the full potential of this dataset. The HHS deserves praise for improving both the quality and transparency of this data over summer and fall, and hope that a broad set of data users—including news organizations, healthcare systems, local government authorities, and public research institutions—can benefit from these new insights into the path of this pandemic.
Note: The maps do not include facilities that report over 120 percent occupancy. Percent ICU occupancy numbers only include staffed ICU beds occupied by adult patients with a confirmed or suspected COVID-19 case.
Source: U.S. Department of Health & Human Services
Graphics by Duy Nguyen and Júlia Ledur
12/12/2020: We have updated map visualizations in this piece to remove what appears to be a data reporting anomaly in a Louisville, KY hospital and to correct the display of all facilities with current COVID-19 hospitalizations in the most recent reporting week.
1 The maps in this piece do not include facilities that report over 120 percent occupancy or fewer than 4 currently hospitalized COVID-19 patients or that appear to have significant data anomalies. Percent ICU occupancy numbers include only staffed ICU beds occupied by adult patients with a confirmed or suspected COVID-19 case.

Dave Luo has an MD/MBA and is a Data Science and Data Viz lead at The COVID Tracking Project.
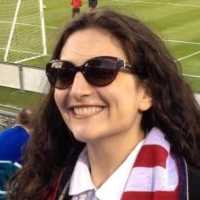
Rebecca Glassman has a Master’s in Public Health and is a public health researcher in academia. Opinions expressed are her own.

Betsy Ladyzhets is a Research Editor at Stacker and works on data quality and the COVID Racial Data Tracker at the COVID Tracking Project.

Catherine Pollack is a third year PhD candidate in the Quantitative Biomedical Sciences program at Dartmouth College. Her dissertation research combines data science, epidemiology, and public policy to combat online health misinformation.
Related posts
What We've Learned About the HHS’s Hospitalization Data
Despite a rocky beginning, the current hospitalization and new admissions metrics from the HHS Protect public dataset have stabilized—and they’re now largely harmonious with state-reported hospitalization metrics if we account for differences in data definitions and reporting lag time.
Hospitalizations Break 100,000, Holiday Data Stalls Out: This Week in COVID-19 Data, Dec 3
Three of our four topline COVID-19 metrics are still recovering from data interruptions over the Thanksgiving holiday weekend. Meanwhile, hospitalizations are soaring.