For a year, The COVID Tracking Project compiled state and territorial data from jurisdictional COVID-19 dashboards. On our website, that data was organized into 32 standardized API categories, corralled into neat charts and tables, and updated each day. But that’s not how the data came to us: In the absence of national COVID-19 data standards, states and territories defined and presented their COVID-19 data in 56 different ways. Harvesting data from 56 dashboards, in turn, created 56 opportunities a day for late or missed updates, for anomalous spikes and drops, and for new or disappearing metrics.
To handle the variability of state COVID-19 data, we developed systems like source notes and data annotations to process where each states’ metrics fit in our API. To handle the data’s unpredictability—the daily delays, anomalies and data dilemmas—we developed an internal state data log where we kept daily notes on every metric we tracked. Today, as part of our data archiving and wind-down, we’re releasing that log publicly for the first time.
What’s in the log
The data log contains a structured record of notes on each COVID-19 data point we tracked, indexed by state, date, and its COVID Tracking Project API field. Those notes represent both developments on the state side, like data notes provided by states, new or disappearing metrics, or missed updates. They also include notes on the COVID Tracking Project’s side, like historical revisions to the data or changes in our API logic.
We started regularly updating the log on July 22, 2020. Before then, we did not regularly track state data anomalies in a structured format; however, the “Data Sources and Notes” section for each state on our Data page contains basic notes on anomalies prior to that point. Meanwhile, our GitHub Issues repository contains a thorough record of any changes that we made to the data.
Over the next few months, you may notice that the log is still expanding. That is for two reasons. First, we are still making some data revisions. We are not adding any newly-released data or making large changes, but we are ensuring that consistent principles are enforced throughout the dataset and any lingering data entry errors are corrected. For example, we recently cleared New York’s cumulative hospitalizations because the data had never been reported explicitly by the state, but we had inferred it from various calculations in early months of data collection, and our policy has been to exclude non-explicitly reported values in our API.
Second, we are making an effort to port data notes before July 22, 2020 from our website, Slack, and GitHub into this log so that our data archive contains a centralized and thorough record of state data anomalies and of our data decision-making.
What we used the log for
The data log was essential to solving one of the biggest challenges of a distributed data project like ours: information sharing around the project. Each day, a different volunteer would lead our data entry team in data collection, and to do that job, they needed the context of what had happened the previous day: whether a state had missed an update, if we were expecting a dashboard redesign, or whether we had started capturing a new metric in a state. For that context, they could check the log.
The log also helped us responsibly caveat the data on our website and social media. We used the log to share any problems that had arisen on our daily data collection to our science communication and data visualization teams, and figure out what needed to be provided as context in our data analysis posts and as labels on our charts.
Finally, we used the log to guide our data capture decision-making. Having a structured record of data notes allowed us to understand patterns in problems for states—for example, whether we needed to put a state on a one-day lag because we frequently missed their data updates—and keep records of the thinking behind historical decisions we had made in handling the data.
How to access the log
To access the log, you can visit our new Data Log page in the About the Data section of our website. From there, you can access the log in Airtable or download it in CSV format. The embedded log on that page will update live as we continue to add log entries.
Acknowledgments
Thank you to Quang Nguyen for creating the first version of the data log and Daniel Lin for developing the second version of the log. Julia Kodysh and Zach Lipton created the infrastructure to integrate the log into our database.
Data quality contributors—including Jesse Anderson, Jennifer Clyde, Amanda French, Alice Goldfarb, Jonathan Gilmour, Matt Hilliard, Hannah Hoffman, Elliot Klug, Camille Le, Brian Li, Daniel Lin, Michal Mart, Theo Michel, Quang Nguyen, Brandon Park, Rebma, Kara Schechtman, and Anna Schmidt, Ryan Scholl, and more—kept our data clean by patching data issues and explaining changes in data log entries and on GitHub.
Data entry shift leads—including Sonya Bahar, Carol Brandmaier-Monahan, Jennifer Clyde, Rebecca Glassman, Hannah Hoffman, Jennifer Firehammer, Amanda French, Pat Kelly, Elliot Klug, Betsy Ladyzhets, Camille Le, Brian Li, Daniel Lin, Michal Mart, Quang Nguyen, Brandon Park, Kathy Paur, Prajakta Ranade, Rebma, Kara Schechtman, Ryan Scholl, and more—submitted daily notes on state COVID-19 data to the log and responded to the daily onslaught of data dilemmas with grace and precision.

The COVID Tracking Project is a volunteer organization launched from The Atlantic and dedicated to collecting and publishing the data required to understand the COVID-19 outbreak in the United States.
More “Testing Data” posts
How Probable Cases Changed Through the COVID-19 Pandemic
When analyzing COVID-19 data, confirmed case counts are obvious to study. But don’t overlook probable cases—and the varying, evolving ways that states have defined them.
20,000 Hours of Data Entry: Why We Didn’t Automate Our Data Collection
Looking back on a year of collecting COVID-19 data, here’s a summary of the tools we automated to make our data entry smoother and why we ultimately relied on manual data collection.
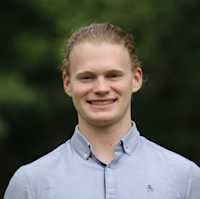
A Wrap-Up: The Five Major Metrics of COVID-19 Data
As The COVID Tracking Project comes to a close, here’s a summary of how states reported data on the five major COVID-19 metrics we tracked—tests, cases, deaths, hospitalizations, and recoveries—and how reporting complexities shaped the data.
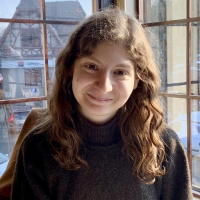
