Over the past year, The COVID Tracking Project relied on state websites to compile a national snapshot of the pandemic. But what we encountered was messy, because each dashboard worked differently. With little guidance from the federal government, states were left to make their own decisions about how to compile and present the numbers. This lack of cohesion led to sweeping inconsistencies across the country, which made it difficult to produce national summaries of COVID-19 statistics and compare situations between states.
Given the extreme fragmentation of the country’s public health infrastructure, there is no easy solution to these problems, and there’s almost certainly no universal fix. In fact, the more we’ve dug into each state’s data reporting pipelines, the more deeply we’ve felt the weight of their individual constraints.
In this post, we’ll cover three classes of problems we identified over our year of collecting and publishing COVID-19 numbers: how data was defined, how data was made available, and how data was presented. As researchers with a national lens, we hope this analysis might help policymakers and health officials to identify common problems with gathering the data and target improvements moving forward.
Data definitions: How do states decide how to count a metric?
Last summer, we wrote about the importance of understanding the varying data definitions behind COVID-19 testing. What we found was that some states were defining their “total tests” number to mean a total count of unique people who had been tested, while other states were using a definition that represented the total count of tests conducted, with repeat testers counted multiple times.
Though this problem has largely been resolved, with the majority of states now counting all tests conducted, many other facets of COVID-19 data are still not uniformly defined. In fact, more than a year into the pandemic, definitions of basic data points—like what even counts as a COVID-19 case—are still not standardized across states. That’s despite the fact that the Council of State and Territorial Epidemiologists (CSTE) has made standardized definitions available.
This brings us to our first class of data problems: States have defined their metrics inconsistently. In some states, too, definitions have changed, or new definitions have been added. For much of the pandemic, for example, Oklahoma was using its disease surveillance system to compile a death count; the death count was a tally of people who were known to have COVID-19 and then died. But at the end of 2020, during a process of reviewing death certificate information, health officials said they had begun to encounter many “incomplete records requiring in-depth investigation,” and in March 2021, the state began publishing a second death metric based on these death certificate reviews.
The public can now see both death counts almost side by side on Oklahoma’s dashboard—one based on disease surveillance and one based on death certificate information.
Definitional differences like these are found all over COVID-19 data, and definitions aren’t always well explained, which challenges any attempt at producing a national summary or comparing situations across states. It’s not always clear whether states are lumping probable and confirmed counts into streamlined total metrics, for example, which might become a problem for people trying to understand the efficacy of testing strategies or how cases and deaths are being identified.
Take the topic of residency, too. Some states have said that their testing, case, and death counts include only people who live in the state, while counts elsewhere include anyone who was tested within the jurisdiction. We’ve seen this again and again—within individual metrics, states have inconsistently divided or merged groups of data.
We’ve also noticed definitional troubles with the country’s long-term-care facility data. Try to quantify COVID-19’s toll on the residents and staff of nursing homes, and you will quickly discover that when states report data by the number of current facility outbreaks, each state uses its own “outbreak” definition. In Illinois, for example, facility names are made public when they have had at least one COVID-19 case within the past 28 days, whereas North Dakota provides a list of facilities that have had at least one case within the past 60 days.
What’s more, a state’s long-term-care data might represent only nursing homes, or it might also include data from assisted-living facilities or personal care homes. And some states combine long-term-care resident and staff numbers, while others report the groups separately.
With race and ethnicity data, we’ve seen huge discrepancies in the categories states track—if information is tracked at all. Alaska reports cases for nine race categories (American Indian or Alaska Native, Asian, Black, Multiple, Native Hawaiian or Other Pacific Islander, Other, Unknown, White, and Under Investigation) and four ethnicity categories (Hispanic, Non-Hispanic, Unknown, and Under Investigation). In contrast, West Virginia reports cases for only four categories: Black, Other, Unknown, and White. Because of these differences, it’s not possible to compile precise national summaries for any race or ethnicity category, nor is it always possible to make comparisons across states.
Additionally, just because a state is tracking certain race and ethnicity categories for its COVID-19 cases doesn’t mean the state will use those same categories for tracking deaths, tests, hospitalizations, or vaccinations. We’ve found that many states have implemented different approaches for different metrics.
Data availability: Is the state making all metrics fully available?
The second class of problems has to do with how states have decided to make their data available.
In Iowa, for example, case data about race and ethnicity is published as percentages, not as raw numbers. According to Iowa’s data, Black or African American people account for three percent of the state’s COVID-19 cases, but Iowa doesn’t explicitly say how many people that three percent represents, nor does the state clarify whether it’s three percent of all people with positive test results or rather three percent of positive test results where information about race was provided. There just aren’t any raw numbers that might help to provide context. (Another 36 percent of Iowa’s cases are listed as “unknown or pending investigation,” but there are no raw numbers available.)
What’s more, Iowa’s race data percentages are reported as whole number percentages, not with decimals, so three percent might in fact be 2.6 percent rounded up or 3.4 percent rounded down—a range so wide, it might distort the public’s understanding by some thousands of people.
And Iowa’s not alone. In data reporting across the country, we’ve found that decisions to round or approximate the numbers not only make it difficult for researchers to analyze the data, but they can also dehumanize the statistics. The lived experiences of real people can become clouded or even erased when precise numbers go unpublished.
For long-term-care data, there has been no consistency in how state health departments have published COVID-19 numbers. Some states provide granular data, showing exactly how many residents and staff have tested positive for COVID-19 at each facility, while other states provide only state-level summary counts. Still to this day, Arizona does not provide any data about COVID-19 at long-term-care facilities other than the number of facilities affected, and New York publishes data about deaths in long-term-care facilities, but not cases.
In nine states, data about COVID-19 cases and deaths in long-term-care facilities hasn’t actually been published on state dashboards. We’ve been able to report these numbers only because health officials sent them to our long-term-care reporting team. There is no valid reason for health officials to opt out of publishing crucial public health data, choosing instead to email the numbers to a non-governmental organization, which then makes the data fully public.
Finally, understanding what happened when is a crucial part of analyzing public health data, and with so many delays and known holes in data pipelines, it is important for numbers to be put into perspective and for anomalies to be reported with context. Throughout our year of data collection, however, we’ve regularly run into trouble gaining access to historical data.
Data presentation: How are provided metrics shown?
For more than a year, we’ve seen states struggle to consistently and clearly present numbers, which brings us to our final class of data problems: how states have set up their websites to present COVID-19 data.
Oklahoma’s situation—with two different death counts displayed almost side by side on the state’s dashboard—can be considered an act of transparency, and it reflects the fact that the CDC itself acknowledges two different methods of counting COVID-19 deaths. But it may not be clear to the public why these two numbers are so starkly different or why the state reports two death counts at all.
Just as it’s important for health officials to think through how and why they are displaying each metric, it’s also crucial for officials to communicate known data delays. Oklahoma’s two death counts were off by nearly 3,000 deaths for a period in late March and early April 2021, with the newly added death certificate count higher than the disease surveillance count. On April 7, the state added a backlog of 1,716 deaths to its disease surveillance count and said that data reconciliation work is ongoing, so additional increases are expected.
Over the course of the year, we’ve repeatedly seen states struggle to communicate these death reporting backlogs and expected delays. Design decisions that fail to signal where data is known to be incomplete have perpetuated the false notion that a state’s daily death report represents the number of people who died that same day, when the process of recording deaths simply takes time.
It’s important for states to be upfront about backlogs, because backlogs distort a real-time view of the data and require the public to shift their understanding of what occurred in the past. Even when states have communicated large backlogs with prominence and clarity, as Alabama did just weeks ago, we’ve seen the immediate effect of numbers being misinterpreted. It is crucial for states to work with journalists and public health researchers to accurately communicate context about the data, and it is crucial for journalists and public health researchers to proactively seek out and explain this context.
Other data presentation bumps we’ve run into might seem like minor inconveniences but pose serious accessibility problems. We remain totally befuddled, for example, as to why New Jersey’s website prevents users whose computers are set outside of an eastern time zone from viewing hospitalization numbers. And across the country, again and again, we’ve seen states require users to toggle hard-to-reach buttons or hover over hard-to-access charts. Design decisions like these can make it tremendously difficult for users to access the data.
Our team of researchers put together this list of recommendations for a national pandemic dashboard last fall.
Our hope: better data standards for the next crisis
This past year, many people have looked to us as an organization that collected and published COVID-19 data, but the fuller story is that working to understand the numbers was just as difficult and involved. Every day, our researchers pored over data dictionaries, read data logs, navigated pesky websites, monitored press conferences and social media pages, and reached out to state health officials. This research provided critical insights to our dataset, and we’ve done our best to communicate the specific differences in how states report these numbers.
We were humbled to do this work. But it is our most deeply felt hope that the next time public health data plays this profound a role in our lives and our policies, it might be a little smoother to unravel and a little easier for the public to follow along.
Jennifer Clyde, Artis Curiskis, Rebecca Glassman, Alice Goldfarb, Michal Mart, Theo Michel, Quang Nguyen, Kara Oehler, Jessica Malaty Rivera, and Kara Schechtman contributed to this report.

Sara Simon works on The COVID Tracking Project’s data quality team and is also a contributing writer. She most recently worked as an investigative data reporter at Spotlight PA and software engineer at The New York Times.
More “Testing Data” posts
How Probable Cases Changed Through the COVID-19 Pandemic
When analyzing COVID-19 data, confirmed case counts are obvious to study. But don’t overlook probable cases—and the varying, evolving ways that states have defined them.
20,000 Hours of Data Entry: Why We Didn’t Automate Our Data Collection
Looking back on a year of collecting COVID-19 data, here’s a summary of the tools we automated to make our data entry smoother and why we ultimately relied on manual data collection.
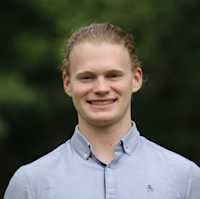
A Wrap-Up: The Five Major Metrics of COVID-19 Data
As The COVID Tracking Project comes to a close, here’s a summary of how states reported data on the five major COVID-19 metrics we tracked—tests, cases, deaths, hospitalizations, and recoveries—and how reporting complexities shaped the data.
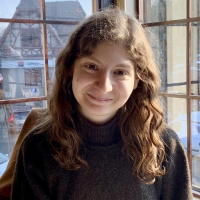
