By the time COVID-19 hit the US, decades of neglect had left our infectious disease surveillance systems fragile, fragmented, and severely underfunded. Nearly a year into the ongoing crisis, health departments are still largely working within the confines of these fractured reporting systems. States still struggle to get complete and timely data from laboratories, and laboratories still juggle byzantine reporting regulations bespoke to each state. Despite a dire need for the immediate transfer of laboratory test results to state health officials, some key reporting systems still rely on faxed paperwork.
Despite these immense difficulties, public health workers across the country have managed to wring critical COVID-19 numbers from aging and inadequate data systems (almost) every day. But the partial success of these efforts obscures a messy reality: Although they’re “working” in the sense that data is flowing through them, these systems are prone to inconsistencies and omissions—which means that some of the data governments use to make highly consequential policy decisions is deeply flawed. This is particularly true with calculated metrics, like test positivity, where data from different pipelines are combined to produce a metric that some jurisdictions use to set travel restrictions, make decisions about reopening or closing schools and businesses, and determine state and county risk levels for public health response. Test positivity has in some cases been so compromised by these data pipeline problems that decision-makers have very likely made consequential decisions based on false signals.
Even when test positivity data comes from federal sources, many of the pipeline woes we’ve uncovered don’t simply go away. Problems travel the course of the pipeline, silently wrecking even the most careful calculations.
We have previously warned that test positivity calculations are fragile and potentially misleading. Our research now suggests that the problems are more widespread, more complex, and more severe than most organizations working with these numbers may realize. In this article, we’ll lay out the scale and scope of the problems we’ve found, and we’ll offer evidence to suggest that policymakers should use these calculations only as rough indicators of trajectory, rather than highly precise benchmarks.
Test positivity: still important, still extremely challenging
A recap for people who haven’t been following the test positivity conversation: Since last fall, we have been investigating the effect of incomplete data on a calculation that has served as a cornerstone of public health policy: test positivity, or a given region’s number of positive tests divided by their total tests. Ideally, test positivity packages up two of the most important facets of the COVID-19 pandemic into one simple metric—a region’s viral prevalence and the strength of its testing strategy. It’s a tool that is used to set policy and help people understand the risk in their communities.
But COVID-19 indicators can only be as powerful as the raw numbers fueling them, and in the US, those numbers come with fundamental limitations. We’ve written about problems related to insufficient testing volume and about mismatched test units, but our ongoing research has uncovered even more problems, mostly related to the decentralized nature of public health infrastructure in the US. Reporting of COVID-19 data is distributed across a network of hundreds of uncoordinated data systems. And especially when case numbers are soaring or health departments are stretched thin, the downsides of decentralized infrastructure are especially profound.
With test positivity calculations specifically, we have found that decentralized health data infrastructures pose two major problems:
Data flows at different speeds for different metrics. Although positive test results require immediate action from state health officials, negative test results do not require any public health follow-up. As a result, while positive tests or cases (test positivity’s numerator) tend to be reported at relatively consistent speeds, the reporting of negative tests (the calculation’s denominator) can lag. This difference in speed throws off calculations combining them.
The limitations of reporting systems silently shape the resulting data. Though states have electronic reporting systems that can quickly send data from labs to health officials, some public health data reporting still occurs beyond the scope of these technologies. To control for potential problems, some state health departments are deciding to omit non-electronically transmitted data from their own test positivity calculations.
In this post, we will briefly discuss both of these problems and then touch on the ways test positivity can—and can’t—be used responsibly in light of them.
Problem #1: Timing mismatches
Test positivity is made up of two metrics—cases and tests—that are tracked using different processes in each state. Usually, states extract their case information out of state health department disease surveillance systems, which promptly record each individual with confirmed or suspected COVID-19 for detailed contact tracing, isolation, and recovery. Meanwhile, total test information, especially the reporting of negative tests, is not always given the same attention; it makes its way through a halting process to those surveillance systems with a combination of electronic reporting protocols (called “electronic laboratory reporting,” or ELR), email, and fax.
You can think of COVID-19 data pipelines as lanes on a public health highway. Just as cars drive in separate lanes, data travels through separate pipelines. On their respective lanes, cars move in the same direction. But ultimately, the lanes have no guarantees of moving at the same speed. In an ideal world, data for cases and tests would flow at exactly the same pace, forming an integrated system of public health surveillance that the Council of State and Territorial Epidemiologists (CSTE) has called the “public health data superhighway.” Two cars on different lanes of this advanced public health data highway—one for cases, one for tests—would arrive together at their destination, the test positivity calculation.
Unfortunately, as CSTE identified, US public health data pipelines are more like an inefficient, clogged highway than a sophisticated interstate. Across the country, data does not always flow in sync; negative tests often hit a traffic jam, resulting in a timing mismatch that compromises test positivity. Many states warn about this problem on their dashboards—like Utah, for example, which discloses that while positive results are reported to the department immediately, negative tests may take up to three days to be reported.
This difference in processing speeds arises largely because of understandable differences in priority. The discovery of a positive case triggers a series of events from state health officials, who will need to trace a case’s contacts and check on them during their isolation period. Negative test results require no public health action. For that reason, some states do not mandate the reporting of negative test results, and even the states that do may not enforce compliance. If labs are reporting via fax or other formats requiring the state health department to do manual electronic data entry, it can be infeasible to count all negative test results, which vastly outnumber positive ones. And when case volume becomes overwhelming, some state health departments have told labs to pause sending negative test results because they don’t have the capacity to process them.
But even when states pick up all test results, they often don’t get positive and negative results at the same time. Most states report cases daily, but not all are able to keep that pace for reporting tests. Even at the national level, cases are faster to recover from holiday reporting effects than are total test numbers. And our researchers have noticed that data dumps of backlogged numbers are far more often backlogs of tests—not cases.
These timing discrepancies between cases and tests can create hitches in test positivity calculations. If someone tries to calculate test positivity on a day when cases were reported by a state, but negative tests were not yet processed, they might get a test positivity of 100 percent. And if they calculate test positivity a few days later, when a backlog of unprocessed tests are dumped into the denominator, they might get an artificially low test positivity. This mismatch in cadences between these different pipelines means that dividing numbers for cases and total tests may not return a useful metric for understanding the impact of the pandemic.
Problem #2: Not every test makes it into total test counts
Differing speeds aren’t the only problem we’ve identified in case and test pipelines. Another sometimes-major difficulty stems from the fact that some COVID-19 pipelines have more intake points than others. As a result, some metrics may include a broader range of data points, while others on the very same dashboard may include only a subset.
Take Arizona, for example. Arizona’s official case count includes individuals whose positive tests were sent to the state health department using a special reporting protocol called electronic laboratory reporting (ELR), fax, or even email. But the negative test reporting pipeline is only set up to count individuals whose results were reported using ELR, so many negative test results will not be included.
Arizona’s own official test positivity number is calculated from a separate set of figures, presented in a buried graph on the dashboard, that subtracts non-electronically reported cases from the numerator. Although this test positivity calculation uses a smaller sample, it more proportionately measures cases against tests, instead of skewing the calculation towards cases. And the difference is dramatic: On February 8, 2021, Arizona’s official all-time test positivity is 5 percentage points lower than one calculated using its public case and test numbers.
Not all states provide the data needed to calculate a test positivity that isn’t affected by these problems. Some states have simply noted that the problems exist without actually controlling for them. And unfortunately, many states simply post test positivity without providing the full underlying data or methodology used to calculate the metric—making it impossible to know whether the calculation is sound.
Federal testing data isn’t immune
After learning so much about problems with calculating test positivity from public state-level data, it’s tempting to assume the federally produced data will fix the problems and allow for clean calculations. To some extent, this is true—test unit mismatches aren’t a problem in federal data, and the testing dataset from the Department of Health and Human Services uses positive tests and total tests drawn from the same feeds for each state, so they don’t have mismatched numerators and denominators caused by separate data sources. Additionally, HHS reports tests by date of specimen collection or date of result instead of date of report, smoothing over data dumps.
But some of these problems are just about whether and when negative tests are being entered into the system in the first place—a problem that affects both state and federal data. When states ask labs to pause reporting negative tests, officials may never be able to backdate the tests in the federal data—either because they never get reported to the department, or, if they do, information about when they were conducted may not be included in the eventual report. And we see signs that this timing problem is affecting the data. Some states still skip updates—as when Washington State couldn’t receive negative testing data in November 2020—and almost all states report dumps of backlogged tests from time to time. That all means that positive tests may enter federal pipelines, which depend on state pipelines, more steadily—and more quickly—than negative tests.
There are also known problems remaining in the federal data, as signalled by a footnote from the HHS dataset noting that five states still cannot submit state-level testing data to the federal government: “ME, MO, OK, PR, and WA test information at the county and state levels is provided directly to the federal government and may underestimate the total number of tests. Because of this, the calculated percent test positivity may be unreliable in some counties in these states.”
Given the significance of the problems we’ve uncovered so far—and the system limitations and inconsistencies that appear to have produced them—we suspect that further problems lie under the surface of federal COVID-19 data.
The most responsible way to work with calculated metrics
That said, there are measures we can take to make our calculations more resilient, like calculating test positivity as a seven-day or 14-day average to smooth out daily inconsistency. And it’s worth acknowledging, too, that imperfect data can still be useful: Treated with the right degree of caution and appropriately smoothed, the data is broadly revealing of trends, particularly within states and at the national level. But the pipeline problems we’ve found suggest that test positivity simply isn’t precise enough to be used either as a strict threshold for policy choices, or to compare outbreaks and testing performance across jurisdictions.
Additionally, for journalists and analysts working with COVID-19 data, we recommend that you:
Use state-provided test positivity metrics instead of attempting to recreate the calculation using public numbers. Do carefully study a health department’s metric, of course, but consider that officials understand the limitations of their own pipelines in ways that those outside the department may not.
Ask questions about where data might be incomplete, and research your state’s data pipelines. We’ve found that systems-level trouble is more likely to be the cause of anomalously high or low test positivity values rather than malicious or deceptive intent.
Remember that problems with data infrastructure can affect the quality of the insights the data can provide. If a total test number has problems, test positivity—even with adjustments applied to smooth the data—silently inherits those problems.
Avoid cross-jurisdiction comparisons that don’t provide context for inconsistencies. State case and testing pipelines are broken in different ways, causing different varieties of data anomalies, and states’ methods for working around those problems may also differ.
For state health officials, we hope you’ll join the ranks of jurisdictions providing thorough documentation of your state’s methods for calculating test positivity—along with any other pandemic indicators featured on your COVID-19 dashboards.
Finally, to all users making calculations using COVID-19 data—not just test positivity, but any metric calculated from it—remember that this data is complicated. It can be used to understand trends, but should not be used in calculations for highly precise thresholds.
Additional research contributed by: Joseph Bensimon, Madhavi Bharadwaj, Jennifer Clyde, Elizabeth Eads, Rebecca Glassman, Michal Mart, Barb Mattscheck, Theo Michel, and Nadia Zonis
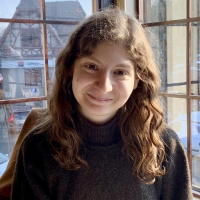
Kara Schechtman is Data Quality Co-Lead for The COVID Tracking Project.

Sara Simon works on The COVID Tracking Project’s data quality team and is also a contributing writer. She most recently worked as an investigative data reporter at Spotlight PA and software engineer at The New York Times.
More “Testing Data” posts
How Probable Cases Changed Through the COVID-19 Pandemic
When analyzing COVID-19 data, confirmed case counts are obvious to study. But don’t overlook probable cases—and the varying, evolving ways that states have defined them.
20,000 Hours of Data Entry: Why We Didn’t Automate Our Data Collection
Looking back on a year of collecting COVID-19 data, here’s a summary of the tools we automated to make our data entry smoother and why we ultimately relied on manual data collection.
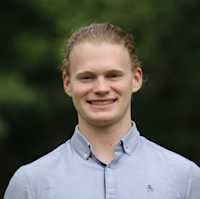
A Wrap-Up: The Five Major Metrics of COVID-19 Data
As The COVID Tracking Project comes to a close, here’s a summary of how states reported data on the five major COVID-19 metrics we tracked—tests, cases, deaths, hospitalizations, and recoveries—and how reporting complexities shaped the data.
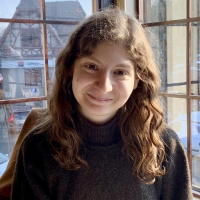
