Since the beginning of the pandemic, there have been a few ground truths about COVID-19 testing data in the United States: It’s messier than it looks, it’s really hard to produce, and it’s very difficult to use responsibly.
That was certainly true back in March 2020, when there was no national source of that testing data at all. In fact, it’s the whole reason The COVID Tracking Project exists. Our project was founded to call state health departments, watch state press conferences, and trawl through 30-page state government COVID-19 reports to answer a single question when the federal government couldn’t: how many tests for SARS-CoV-2 had been administered in the United States?
By summer, federal and state governments had both begun making testing data more accessible. But problems persisted: In state-provided testing metrics, inconsistent units of measure at times made it incorrectly look as if some states were doing a fraction of the testing that others were doing. And when we did an in-depth analysis on the initial federal release of testing data in May 2020, we found that it often diverged dramatically from state total testing data in inexplicable ways.
Unfortunately, the core problems remain. You can get testing data in similar units from all but two states, but you’ll find some states report sporadically and include different kinds of tests in the totals. Federal testing data, too, still has noticeable gaps and mysteries. State-provided total test counts and those posted by the HHS and CDC still disagree for over half of US states.
Most of this post will be dedicated to showing what we’ve learned about the likeliest reasons for these discrepancies. At least for the moment, our findings suggest that the ground truth of testing data has withstood the test of time: Both state and federal testing data are as fickle to work with as ever.
But the findings also lay a clear path for the federal data to finally escape that pattern. With more investment on the part of government, the federal testing dataset can become a high-quality, standardized source of testing data for the United States. Over the next few months, starting with this blog post, we’ll be sharing our diagnosis of the problems the federal government will need to resolve to reach that point based on what we’ve learned about how states report to them.
Before we dive into that analysis, it’s worth noting that the prospects for federal COVID-19 data have improved substantially since we first took a look at it.
First, the data released by the federal government has become much better standardized than state data will ever be. The COVID Tracking Project can only skim the surface of state dashboards in an attempt to stitch together a cohesive testing dataset, but federal agencies receive detailed testing data directly from states and labs. Despite some early missteps, by getting direct access to the underlying data, the federal government now avoids many of the biggest standardization problems we’ve struggled with:
Federal data standardizes units of measure: It took the better part of a year for most US states to both explain how they count tests (in people tested vs. specimens vs. test encounters) and for all but two states to standardize on comparable methods, so our dataset has always included both known and silent unit-mismatch problems. From the start, the federal government has asked states to submit data in units of tests, not people, which largely obviates the unit confusion.
Federal data standardizes test types: In our compiled data, we attempt to separate out PCR tests and antigen tests, but some states do not publish separate totals of PCR tests—or just don’t make it clear what tests are included in the totals they are publishing. Fifteen states publish only a lumped number containing both PCR and antigen tests, and we have been unable to get answers on what test types are included in four states’ reporting. Test results submitted to the federal government include a test type, so there should be no confusion or combining of disparate types in federal figures.
Federal data gets around date-of-report problems: We arrange all the data we compile by date of public report—not by the more epidemiologically correct schemes that arrange data by date of test-specimen collection and require daily updates of the entire time-series to backfill newly reported data points. As a result, if a state processes a big backlog of tests on one day, it will show up as an artificial spike in our data. We have been unable to use more epidemiologically precise dating schemes because not all states offer public historical data on their dashboards. The federal government receives backdated data by date of specimen collection or by date of result each day from states and labs.
Second, the current administration is taking both testing and data more seriously than the Trump administration did. The Biden administration issued an executive order on data-driven response to the COVID pandemic, and in its COVID-19 response and preparedness plan, it specifically lists testing as one of the core data priorities.
And third, even before the transition to the new administration, the federal government took great strides toward making its testing data more complete, more transparent, and more granular. All but 5 states now submit detailed testing data to the federal government, far more than did when we originally analyzed the data. And using that more granular testing data, the Department of Health and Human Services now publishes the daily Community Profile Reports that have guided the federal response throughout the pandemic. Along with making county-level data available to the public for the first time, the report’s data notes explain some of the largest remaining discrepancies between state and federal testing data.
State-level data, by contrast, is largely as fragmented and unstandardized as it was in May: some vexing problems are nearly resolved, but equally profound ones have replaced them. The best hope of a comprehensive national testing dataset now comes from the federal government. Its data should displace the patchworked dataset we’ve relied on from states while national health agencies floundered. If the federal government ignores or further delays addressing the problems in its dataset, it will miss the United States’ only shot at a complete and fully credible testing dataset at a moment when the country still desperately needs one.
Federal testing data vs state-reported public testing data
To understand the similarities and differences between the two datasets, we compared testing data posted by HHS with the data we collect from states’ data dashboards. We were able to compare federal and state data in 52 of the states and territories for which both CTP and the federal government collect data. The federal data includes the Marshall Islands, which The COVID Tracking Project’s state dataset does not, and we publish data for American Samoa, which the federal government does not. Though the federal data has updated totals for Puerto Rico, the territory has not posted testing totals since August, so we excluded it from our analysis. Finally, we excluded two jurisdictions that only post test results in unique people (Alabama and the Virgin Islands) because the difference in units between state and federal data drowns out any other more subtle cause of divergence.
For the states we could compare, we calculated percent differences on January 29 (a date selected to avoid fluctuation from backfilling in the federal data, which is generally concentrated in the most recent few weeks of data) and plotted comparisons of federal and state data going back to March 2020:
First, the bad news: Federal and state testing figures come within 5 percent of each other for only 10 of the 52 jurisdictions we compared.1 Of the remaining 42 states, 17 publicly report testing totals that are lower than the number in the federal data, and 25 states publicly report total test numbers that are higher than the number in the federal data.
We had hoped that most or all of these gaps could be explained by known deficiencies in our compilation of publicly reported state data, but although they may account for some of the gaps, they don’t provide a full explanation.2 We examined state and federal gaps for the three major deficiencies noted above—the different selection of units, the inclusion of antigen tests, and the different dating schemes—with mixed results.
Units of measurement may explain some or all divergence for up to seven of the 17 states that report lower numbers than the federal government does. Six of the states that report lower numbers report publicly in test encounters rather than in specimens. If the federal dataset uses a specimens number, we would expect the federal data to be a bit higher than the state data, because test encounters exclude multiple tests administered to one individual on the same day.
Lumped antigen testing data may account for some or all of the divergence for up to 10 of the 25 states with publicly reported totals that are higher than the federal data for their state.3 Logically, these totals should be higher than those reported to the federal government, which do not include antigen tests, so this factor could explain some or all of the divergence in those states.
The difference in dating schemes does not, on its own, explain the gaps between state and federally reported testing data. Backfilling by date of specimen collection generally only causes differences in the distribution of tests over the time series rather than the total number of tests. The large gaps between these two datasets are in the total numbers of tests ever reported, not just in the days to which those tests are attributed. But to rule out this cause definitively, we also plotted the federal data against backfilled time series provided directly by states. In states where the data was available, using these time series did not ameliorate the problems.
If we assume that units and test types explain all the differences in the 17 states where they apply—which is unlikely—that still leaves 25 states and territories with apparently inexplicable differences between COVID Tracking Project data derived from the states and federal data.4
In all of these states, the definition of total tests appears to be exactly the same, but the number of total tests diverges by between 5% and 79%. If the federal government and a state mean the same thing by “total tests,” then either the state dashboard data or the federal data must be incomplete. Our researchers have found indications that, at least for some states, tests are getting lost in the state pipelines that feed into the federal numbers.
But to understand the ways in which data can go astray between states and the federal government, first you need to know a little bit about how these inputs work.
Meet the federal data systems
The reason COVID-19 testing data is so complicated is that before the pandemic, there was no comprehensive federal system for tracking total test results. It was only in April that the federal government spun up an initiative called the COVID Electronic Laboratory Reporting program—CELR for short—to create a national dataset; since May, the federal government has made that data available to the public.5 CELR isn’t really a single system—it’s a combination of multiple systems, new and old, that the government pieced together to build a testing dataset. Tests enter this network of systems through three main inputs:
Data from commercial, clinical, and public health labs: Six large commercial labs, hospital labs, and public health laboratories submit testing data directly to the federal government. This data picks up on only a portion of tests administered in a state, since not all labs in each state submit data to the federal government.
Aggregate data from state public health departments: The CDC asks states to submit a simple count of how many tests they’ve run each day, broken down by test type (PCR, antigen, serology) and result (positive, negative, inconclusive).
Line-level data from states: The HHS asks states to submit a line-level feed containing details of every test conducted in a state. In line level data, each test is represented as a line in a file—which you can picture like a row of a spreadsheet—which contains details like the test result, the manufacturer and make of test, or demographic information of the recipient. 6
These three streams of data are then compiled into one unified dataset in the new HHS Protect system, with each state’s data drawn from just one of the three streams.7 Of all the options, the federal government prefers to get tests from states at the line level because this method includes a greater level of detail for each test counted; for example, it preserves any demographic information associated with a test and allows for breakdowns of tests by county. For jurisdictions unable to submit line-level data, the federal government accepts aggregate data, which it currently receives from Ohio, Wyoming, and the Virgin Islands.
For states that do not submit usable testing data using either of these routes, the government falls back to the incomplete lab submissions—which is also how it gets county-level data for Ohio and Wyoming (the Virgin Islands submit aggregate county data). Five jurisdictions don’t submit any testing data directly to the federal government: Maine, Missouri, Oklahoma, Puerto Rico, and Washington.8
So what does this journey through federal testing data pipeline woes explain? Not as much as we might like, but it does explain the discrepancies for the five jurisdictions with the highest unaccounted-for differences between federal and state totals: they’re the states and territories for which the federal government uses lab-submitted data. In this group, testing volume reported on state dashboards is between 36% and 79% higher in the state counts than the federal counts.9 This difference is to be expected: The lab-submitted data used by the federal government captures only a subset of laboratories in each state.
One of those five jurisdictions (Oklahoma) is already on our “possibly explained by antigen lumping” list, and one of them (Puerto Rico) is excluded from our comparison because of a lack of recent data, so that means that we have now added three more—Maine, Missouri, and Washington—to the “differences explained” category. This leaves us with 22 states with substantial, unexplained gaps between state and federal data. Those 22 states all submit data directly to CELR—and all use line-level protocols, the federal government’s preferred method of submission. This means that the differences we’re finding between their public dashboards and the federal data reveal a difference between what a state publishes and what that same state submits to the CELR program.
At this stage in our analysis, we should be clear: Past this point, we have no complete answers about the difference between federal and state data, because we haven’t been able to interview each jurisdiction’s public health officers about the gory details of their CELR submission process. What we do have, though, are suggestive clues based on helpful, often highly detailed discussions with officials in a half-dozen representative states who were willing to describe their submission process.
What we’ve learned so far suggests that discrepancies between state and federal testing totals arise because state public health officials, strained for time and resources, sometimes end up counting tests for submission to the federal government in less comprehensive ways than they do for their own dashboards.
Meet the state data systems
All COVID-19 data that arrives in a federal system comes from somewhere else: from labs, from hospitals, and—for the majority of data points—from state public health systems. And as with federal systems like CELR, state public health systems are usually conglomerates of smaller, often rickety systems patched together—including electronic submission protocols, surveys, portals, even faxed spreadsheets—to accomplish the Herculean task of counting every COVID-19 test conducted within a state’s borders.
Each jurisdiction approaches the problem differently, so there’s no way to offer a standard account of how a COVID-19 test report flows into state, then federal, data systems. To understand the discrepancies between state and federal testing data, what we need to know is that the federal government also lacks a standard account of how data gets into their systems. The federal government may ask states to submit data each day using a certain CSV template, but they don’t prescribe standards about how data should get into the cells, nor could they if they wanted to—states wouldn’t be able to build the infrastructure overnight to meet those requirements.
As a result, in each state, tests enter data pipes through multiple intake points—and are extracted by state health departments at multiple outflow points. These different paths are what we believe cause the discrepancies between state and federal testing data: When health officials pull data out of their systems at different points for their dashboards than for the federal government, a different mix and quantity of tests will show up on the dashboards than in the federal systems.
It’s easiest to identify this trend in the simplest cause of divergence between state and federal data, which is when the points of extraction for state and federal data pick up different data sources.
We’ve spoken with one state, for example, which submits data to the federal government by routing only one of their pipelines for collecting test data—their electronic laboratory reporting (ELR) feed—directly to the federal government. ELR is a standard and quick method for transmission of laboratory results that picks up on the majority of test results in most states. But in this state, a small but significant number of laboratory results still flow to health departments through submission methods like faxes, web form, or emailed spreadsheets. Those faxed and emailed results flow alongside ELR data into the state’s centralized disease surveillance system, and from there to state dashboards. As a result, faxed and emailed tests get captured on the state dashboard, but don’t make it to federal systems, leading to double-digit percentage differences.
States submitting data to the federal government out of their surveillance system, instead of from their ELR feeds, can face a related dilemma. In one such state, public health officials told us they haven’t been able to get all their lab results into the surveillance system—especially those coming from nontraditional testing sites, which may come in nonstandard formats. When health officials cannot wrest data into their systems, they manually add them to the total number of tests that they publish to their dashboard. But the federal numbers, which come directly from the surveillance system, miss out on those tests.
We expect that many states with differences between federal and state dashboard data face similar problems. A recent Council for State and Territorial Epidemiologists poll of 44 states found that almost all receive a portion of their data in formats other than HL7 message, ELR’s standard format; just under half receive results from some labs by fax. If these states are submitting just their ELR to the federal government, that would result in federal undercounts of the testing data. Differences in data sources can also cut the opposite way, like if a state chooses to publish only ELR tests on its dashboard, but shares more comprehensive counts with the federal government.
Even if states did manage to get all their sources to feed into the different points in the pipes that produce counts for their dashboards and federal data, the difference in process poses another hazard: Each process for extracting testing data from the pipelines requires continual maintenance. When officials are squeezed for time, maintaining the federal data outputs tends to fall to the wayside.
One state we spoke with doesn’t maintain its list of COVID-19 test codes for which to submit data to federal systems, so federal data for that state is missing results from newly authorized tests. Another state doesn’t reconcile data dumps from laboratories with the data it submits to federal systems, resulting in a federal undercount for that state. Yet another state doesn’t process the data it submits to the federal government to remove accidental duplicate reports of the same test, leading to a federal overcount for that state.10 There are probably dozens of other process quirks leading to mismatches with federal data in the states whose officials haven’t had the time to sit down with us.
The best way to eliminate these discrepancies would be for states to produce both their federal outputs and dashboard data the same way. In the one state we’ve been able to confirm produces both state and federal data out of a centralized system, the totals always come within a few percentage points of each other. But many states don’t have the resources or infrastructure that they would need to do that.
More detailed, less comprehensive
The full story of why most states ended up where they are, using different processes to produce state and federal versions of testing data, would need to cover a long history of underfunding in public health that resulted in the decentralized and disorganized health data infrastructure we have today. But you can get the condensed version of that story by considering the question that every overextended state public health department has had to answer in the past year: What is the fastest, easiest way to get the data out of their overburdened systems to state dashboards and to the federal government?
On their state dashboards, officials want to produce the most up-to-date, aggregate counts of how many tests have been conducted in their state. Dashboards only need a count of tests administered each day—which is why officials sometimes choose to take the unconventional step of extracting counts from files before laboriously transforming the data into a format their official surveillance system can read.
The federal government’s goal is also to publish up-to-date, comprehensive testing data, but it asks for something more than a dashboard requires: highly detailed line-level testing data. To manage submitting data in that format, states may choose to publish out of a less comprehensive system than their official surveillance system, such as their ELR feed.
Both of these deviations from publishing out of the surveillance system can lead to less comprehensive federal data. But we have seen no evidence that the federal government continuously monitors states’ submissions to ensure that the richly detailed data they request also meets the more basic goal of catching all—or most—of the tests done in each state. As a result, it appears that some states considered to have “completed” onboarding to CELR by the CDC are meeting the technical requirement of submitting line level data without fulfilling the overarching goal of submitting up-to-date counts of all their tests.
Aside from all the quality problems we have learned about directly from states, this pattern becomes evident on a large scale if we look at the history of states’ federal testing data submissions. Each day, states have the option to submit historical testing data to the federal government: For example, on February 15, 2021, not only did states tell the government how many tests they ran on February 14, but many of them also submitted results dating back to early 2020. Usually, any revisions submitted by states are relatively small and unrevealing, especially far back in the timeseries. But if we visualize the full history of states’ data submissions, we can see large shifts on key days in some states affecting the differences between state and federal counts:
These charts visualize every day of six states’ federal data submissions. Each gray line represents one day’s submission. Usually, it’s difficult to distinguish one day’s submissions from another’s since they are so close to each other. But some states have days where the curves shifted dramatically, and in the six states pictured, those shifts are on significant dates: They correspond to the window of time that the federal government switched to these states’ line-level submission instead of aggregate or lab-provided data.11
Alongside the historical submissions, we plotted data from state dashboards in blue. As the comparisons demonstrate, switching to line-level testing data doesn’t guarantee that the gap between state and federal test counts will shrink. In some cases, that switch appears to have made that gap worse.
The historical submissions of some states in these charts that likely used aggregate submission before they switched to line-level data—such as California and North Dakota—start out matching closely, but they fall out of sync once the state switches to line-level submission. Other states—like Delaware or New Mexico—improve substantially after an initial backfill, likely because the federal government was initially relying on direct submission from labs. But their line level data then falls out of sync with the dashboard again, probably signalling a lack of maintenance of the line-level data submissions. In all cases, differences that start small grow large over time—both because small daily differences compound and, in some cases, because daily differences grow at increasing speeds as federal submission pipelines fall into disrepair. This suggests that the federal government may not be continuously checking that states’ submissions are comprehensive after validating the initial switch.
We cannot see these shifts to line level submission in all states, sometimes because we couldn’t track down their CELR adoption data, sometimes because we couldn’t see any major backfills in the public history of data submissions (which goes back to late July 2020). But these six comparisons of the historical federal submissions to state data suggest that the CDC’s main criterion for high-quality testing data—detailed, line-level data submission—does not necessarily guarantee a comprehensive federal count of a state’s tests. In fact, it may even work against that basic goal of a comprehensive count.
Why—and how—to improve federal testing data
The federal government undoubtedly intends to capture testing data that is both richly detailed and comprehensive, and this is unquestionably the right goal. If the federal government gives up on collecting line-level data, the United States will never have the data it needs to implement a truly informed national testing strategy. If, on the other hand, federal agencies accept substantial undercounts (or overcounts) of total tests as the price of receiving more granular data, those agencies will lack even a basic knowledge of how many tests are being done in each state.
To ensure that states can submit data that is both comprehensive and richly detailed, the federal government needs to provide more support to state public health departments. This is also a question of equity: Right now, only the nation’s best-funded state health departments can afford to send good data to the federal government. And even within states, the labs that submit the worst data are usually those with the oldest data infrastructure, which means county-level disaggregations within a state may vary in quality along socioeconomic lines. Good federal testing data is currently a matter of haves and have nots, which means that data-driven federal policy is necessarily also uneven.
To create a comprehensive, reliable national testing dataset—one that can support an equitable pandemic response—the federal government should take on three immediate goals:
Bring the five remaining states not using CELR into CELR as soon as possible: Data from states where the federal government relies on its own network of laboratories is so incomplete as to be unusable.
Invest in consistent, high-quality state submissions: Our research suggests that the CDC validates state line-level submission when states initially begin submitting to CELR, but that it allocates far fewer resources into continuously assuring the quality of these feeds. As a result, gaps between state and federal data often compound over time. Federal public health agencies should continuously work with states to ensure they follow best practices in their test data submission and provide states with the resources they need to make that happen.
Do everything necessary to make CELR data the gold standard: Right now, most state public health departments use their internal analyses, not federal ones, in their public data outputs. As a result, the data they submit to federal systems is often less comprehensive and less carefully maintained, leading to major gaps in the federal data. The United States deserves a trustworthy central testing dataset as a national reference point, and the only way it can achieve that goal is if the CDC and HHS can collaborate with states such that states prioritize—and trust—the data they submit to federal systems as highly as they do their own data.
This work will be difficult—and assembling COVID-19 testing data is already difficult. It is hard for staff in nursing homes and schools to enter testing data into convoluted web portals each day, hard for exhausted state officials to figure out how to import the latest non-standard CSV from a new testing site into their data systems, and hard for the overburdened federal agencies that need to monitor 55 different, intricate state pipelines.
Counting tests is so hard, in fact, that some prominent voices have floated the idea that we stop doing it. This would be a disaster: Case, hospitalization, and death data can tell us what the pandemic is doing to us, but only the number of tests we’ve performed, along with the number of vaccinations administered, can tell us what we’re doing about the pandemic. When we let testing data slide, we can’t tell how well we’re responding to outbreaks—or if we’re responding at all.
We don’t think it’s very likely that the federal government will stop collecting testing data altogether. We do think that it’s very tempting to let the federal dataset’s quality problems persist in the face of other pressing priorities.
But we also expect that the new administration, with its demonstrated understanding of the crucial role testing will play in defeating this pandemic, will be willing to resist this temptation, and instead make a substantial investment in creating a genuinely trustworthy and comprehensive dataset that can guide a credible and comprehensive response. And we believe that, when it does, the patchwork testing dataset our project was founded to create will finally be what we’ve always wanted it to be: obsolete.
Additional research and contributions from Alexis Madrigal, Dave Luo, Erin Kissane, Michal Mart, Peter Walker, Rachel Glickhouse, Ruirui Sun, and Theo Michel.
1 Differences below 5% are most likely due to the different publishing cadence of federal and state data.
2 The federal data also includes inconclusive tests, which some jurisdictions’ testing totals exclude. However, these tests account for 0.2% of total tests in the HHS data on average, so they could not be a significant contributor to discrepancies. The one exception is Oregon, where inconclusive tests make up 2% of the federal totals and inconclusive tests are not included in state totals, but this factor alone still cannot explain the 7% discrepancy between federal and state data.
3 We have categorized states as lumping (or not lumping) antigen tests based on notes on their dashboard, our outreach to state health departments, and external reporting. One state, West Virginia, says it reports PCR tests on its dashboard, but media coverage has said it includes antigen tests as well; we count it in neither group for this reason. In this group of 25 states and jurisdictions, we have not been able to find any information about Puerto Rico’s test totals. The remaining 14 states with totals higher than those of the federal government appear to include only nucleic acid amplification tests.
4 There are also reasons to believe that antigen lumping and test units cannot fully explain the difference between state and federal totals, even in the states where these problems are present. Though antigen testing is relatively common by now in the US, antigen reporting is still extraordinarily spotty; it would be surprising if states were picking up on enough antigen tests to the point that the federal totals were substantially lower than those on those states’ dashboard just because of antigen tests’ inclusion in the latter. Meanwhile, while counting tests in units of encounters as opposed to specimens makes a difference—as we know from the states that have posted test counts in both units or switched from one to the other—these differences are relatively small. It is uncommon at this point in the pandemic for an individual to be administered two tests in one day, a measure that was more frequently taken early on as duplication to control for false negatives. As a result, counting individuals tested per day and counting specimens tested yields similar numbers.
5 Fun fact: CELR is so new that state and federal health officials haven’t yet decided how to pronounce its name: we’ve heard “cellar,” “sealer,” and “C.E.L.R.” so far.
6 If you’re interested in all the fields the federal government asks states to submit, you can check out the HHS’ laboratory guidance, first issued in June 2020.
7 Though the CDC publishes what method each state uses to submit to CELR on a webpage, it has not been updated since November 2, 2020. The most up-to-date source of this information is the Data Notes section of the HHS’ daily Community Profile Reports Excel download.
8 On its website, HHS says the only reason it would use its own lab dataset is for states that cannot separate their serology and antigen test results from their PCR test results. Puzzlingly, three of these five states have already demonstrated this capability in their public data releases: Maine and Missouri by disaggregating tests by type on their dashboards, Washington by only posting PCR tests on its dashboard. It is unclear why the federal government is using lab data for these three states.
9 In Puerto Rico, the January 29 federal totals are 16.3% lower than the most recent total test number we have received from the state. However, CTP’s most recent state-sourced testing figure for Puerto Rico is from August 28, 2020, whereas the federal government last received data from the territory on January 30, 2021. Even if Puerto Rico includes antigen tests in their data, the federal totals should be substantially higher than the CTP values since they capture nearly half a year more testing volume; that they are this much lower signals an especially substantial data completeness problem.
10 The federal government also processes testing data to remove duplicate reports, which occur when facilities submit testing data for the same test (for example, if both a university and its contracted lab submit data to the state) or when a state accidentally uploads two files. But federal processes catch fewer of these duplicate reports because the data it gets are de-identified; where state algorithms can detect duplicate submissions by using information like names associated with the tests, federal algorithms cannot.
11 Where we were unable to confirm the date of a state’s CELR adoption by other means, we estimated them from archives of past CDC “COVID-19 electronic laboratory reporting implementation by state” reports. There were 8 reports we could find, published between July 17 and October 26, 2020. All reports presented a table with the “Line Level Onboarding Status” for each state. If a state’s status changed from “In progress” to “Completed” in 2 consecutive reports, we decide its line-level CELR adoption happened within the timeframe of the reports’ publication dates.
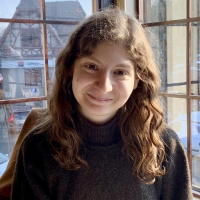
Kara Schechtman is Data Quality Co-Lead for The COVID Tracking Project.
More “Testing Data” posts
How Probable Cases Changed Through the COVID-19 Pandemic
When analyzing COVID-19 data, confirmed case counts are obvious to study. But don’t overlook probable cases—and the varying, evolving ways that states have defined them.
20,000 Hours of Data Entry: Why We Didn’t Automate Our Data Collection
Looking back on a year of collecting COVID-19 data, here’s a summary of the tools we automated to make our data entry smoother and why we ultimately relied on manual data collection.
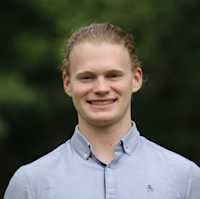
A Wrap-Up: The Five Major Metrics of COVID-19 Data
As The COVID Tracking Project comes to a close, here’s a summary of how states reported data on the five major COVID-19 metrics we tracked—tests, cases, deaths, hospitalizations, and recoveries—and how reporting complexities shaped the data.
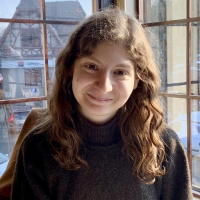
