Although The COVID Tracking Project has ceased data collection, we’ve published a series of explainers about federal COVID data and held trainings on how to use that data. More federal data has been released nearly every week this month, and we remain confident that the federal data ecosystem will continue to improve. We’ve also noticed that over the past few weeks, newsrooms of all sizes—and even some government agencies—have fallen into some of the data potholes that we’ve become extremely familiar with in our year of wrangling public COVID-19 data, so we’re offering a brief cheatsheet on avoiding some of the most common errors we’ve seen.
If you see dramatic movement in the data, look for contextual clues before interpreting it as a change in the pandemic
COVID-19 data is messy and complex—especially if you’re working with data arranged by date of report (instead of by date of test-specimen collection or symptom onset or actual date of death). To avoid drawing conclusions that are too broad about the pandemic, watch out for confounding factors, which we discuss in detail below.
Day-of-week effects in data arranged by date of report produce predictable reporting swings over the course of each week. Every metric behaves a little differently over weekends, and it’s important to account for these artifacts—especially because they can stack onto other reporting irregularities and produce data changes that look very substantial. We’ve written a whole post about this issue, and encourage analysts to rely on seven-day averages or weekly views of the data to reduce confusion.
Data backlogs—and the “data dumps” that occur when those backlogs are resolved—can mimic major declines and then jumps, especially in cases, tests, and deaths. These irregularities can produce erratic drops and rises in the numbers. We recently saw a national newsroom report that a state had seen a 90% increase in COVID-19 cases, when in fact the state had merely experienced a data dump of historical case counts, which was noted publically. We also watched as several data users and newsrooms made incorrect national conclusions about deaths after one state processed an enormous amount of death certificates from the winter. We advise data-watchers to always look for official explanations on state dashboards before assuming that a jump or drop in a single state’s numbers reflects the reality of the pandemic. Newsrooms should go a step further and contact the state’s public health department when they encounter unusual patterns in the data.
Holiday (and weather-related) reporting issues happen when national or natural events occur across many states at once, and can mimic shifts in the pandemic. For broader regional or national trends, look for nearby holidays or other major disruptions like regional power outages or storms that might have artificially depressed—and then inflated—the data. Clues that an anomaly may be behind a national or regional movement in the data include a simultaneous drop or jump in both cases and deaths (in reality, reported deaths lag behind reported cases by several weeks) and stable hospitalization numbers. But even hospitalizations—our sturdiest metrics through holidays and storms—are affected to some degree by holidays and disruptive weather, both because of reporting problems and because of behavioral changes in people seeking and accessing care.
Watch out for definitional mismatches and alternate dating schemes
Different jurisdictions chose different ways of defining and reporting their metrics. This makes things like test positivity so complicated that we’ve written three blog posts about the perils of comparing this calculation across states and territories. It can also complicate comparisons of more straightforward metrics like deaths or hospitalizations. Federal data has standardized some but not all of these inconsistent data streams, but when analyzing differences between states, it’s still important to look for definitional differences—even in federal numbers.
Data arranged by standard epidemiological dating schemes—date of symptom onset, date of death, etc.—enable more precise analysis and remove many of the reporting hiccups we discussed above, but these methods come with their own downsides. Because each day’s newly reported numbers have to be painstakingly assigned to their correct date in the past, data arranged in this way is constantly in flux as new historical data points come in and get reconciled into their correct date.
Get familiar with caveats
Even more importantly for the casual observer, the most recent dates in epidemiological datasets are always incomplete—because, for example, the data points for people who died today won’t finish being reported for many days, weeks, or even months in the future. As a result, the most recent data always drops to zero, no matter what is actually happening in the movements of the pandemic. We’ve seen visualizations of these datasets become a source of honest confusion as well as tools for intentional misinformation about COVID-19 in the US, and urge caution in their use with a general audience.
Be cautious about what the data can say
Over the course of the pandemic, we’ve seen many officials make claims about the causes of changes in the pandemic. If you’re trying to extract insights from the data itself, it can be very easy—especially within a headline—to make causal claims when only correlative evidence is available. Case spikes after holidays might be related to large gatherings and travel, or they might be entirely explicable by the reporting problems we outlined above. The same applies to drawing conclusions on public health mitigation policies based on how the trend lines are behaving. Public health officials often have access to non-public data from case investigations that can help them interpret the topline metrics, but we recommend that reporters and members of the public take a conservative approach to claims and avoid the fallacy of causation without evidence.
Throughout the pandemic, we’ve argued for the need to discuss COVID-19 data in ways that are clear, transparent, and honest. While it may make for less pithy headlines, including caveats and disclaimers with your data conclusions will allow for much better understanding of this complex pandemic.
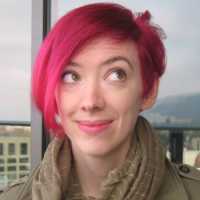
Erin Kissane is a co-founder of the COVID Tracking Project, and the project’s managing editor.
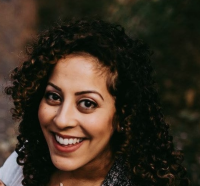
Jessica Malaty Rivera has an MS in Emerging Infectious Diseases and is the Science Communication Lead at The COVID Tracking Project.
More “Federal COVID Data 101” posts
Federal COVID-19 Testing Data Is Getting Better
The federal government improved its state and county-level COVID-19 PCR testing data since we analyzed it in February. Here’s an update on those changes and what we hope to see next for the data.
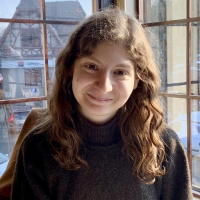
Federal COVID Data 101: What We Know About Race and Ethnicity Data
Publicly available federal race and ethnicity COVID-19 data is currently usable and improving, although it shares many of the problems we’ve found in state-reported data.

Federal COVID Data 101: How to Find Data
Here’s where and how you can find COVID-19 data now that The COVID Tracking Project has ended.
