To understand any dataset, you have to understand the way its information is compiled. That’s especially true for a patchworked dataset like US COVID-19 data, which is the product of 56 smaller systems belonging to each state and territory in the country.
In our year of working with COVID-19 data, we harnessed our attention on these systems and found that the data they produced often reflected their individual structures. This reality runs parallel to the country’s biggest public health data challenge: the data pipelines that so deeply affected the trajectory of the pandemic were not given the decades of support—financial and otherwise—needed to perform well under pressure. Instead, a novel threat arrived, and the data response we saw was fragmented, unstandardized, and limited by the constraints of the systems.
In this post, we’ll offer a summary of how states reported the five major COVID-19 metrics—tests, cases, deaths, hospitalizations, and recoveries—and a look at how reporting complexities shaped our understanding of the pandemic. We’ll also link you out to in-depth resources, both on our own site and others, on the reporting of each metric.
Tests
Before the COVID-19 pandemic, the Centers for Disease Control and Prevention had never collected comprehensive national testing data for any infectious disease in the United States. But in March 2020, as COVID-19 began to spread throughout the country, the number of tests conducted became the most critical data point to understand the pandemic. Without it, we couldn’t understand if and where low case counts were just an artifact of inadequate testing.
So, in April 2020, the CDC partnered with the Association for Public Health Laboratories (APHL) to start the COVID Electronic Laboratory Reporting Program (CELR), which would eventually collect detailed COVID-19 testing data from every state. While the federal government and APHL onboarded every state to CELR, which took just over a year, The COVID Tracking Project stepped in to compile a national testing count from state health department websites. Like the CDC, states had never collected data at the scale the pandemic demanded, and as a result, all testing data was incomplete and unstandardized.
The pandemic exposed the extent to which the United States’ critical but chronically underfunded laboratory data infrastructure was at the mercy of the fax machine, with manual data often failing to make it into state counts or causing distortionary effects like data dumps. In addition, as nontraditional settings like schools and nursing homes started administering antigen tests, states lost sight of how many of these COVID-19 tests had been administered—opening a hole in our understanding of US testing volume as antigen testing took off in the fall of 2020. Laboratories unaccustomed to collecting demographic data often failed to collect information on the race and ethnicity of those seeking COVID-19 testing, even though federal guidance required it.
The way states reported testing data was dictated by these difficulties they faced in collecting it, and because each state had slightly different weak spots, reporting was unstandardized. Some states reported just electronically-transmitted lab results, while some reported faxed data too. Some states reported antigen tests (or early on, antibody tests) combined with PCR data, some separated them out, and some states didn’t report them at all. Race and ethnicity data was highly incomplete and unstandardized, impeding efforts to understand the disproportionate effect of the pandemic on Black, Latinx, and Indigenous communities.
Of all the inconsistencies across states, one extraordinarily daunting problem that did improve over the course of the pandemic was the variation in testing units. For much of the pandemic, some states chose (or only had the capability) to count the number of unique people tested rather than the number of tests conducted. Because individuals are likely to receive multiple tests for COVID-19 over time, states counting people rather than tests appeared to be doing much less testing than others, throwing off measures used to contextualize case counts, like test positivity. By the end of our data collection, all but two jurisdictions had standardized on counting tests rather than people—although there are still some variations within how states count tests.
Only the CDC ever stood a chance at collecting testing data that was standardized across jurisdictions. But the federal government has faced its own share of problems in putting together a national testing dataset. When federal testing data was first published in May 2020, many states still had not started submitting data to CELR, leading to a dataset that was highly divergent from state data because it had different sourcing. And even now, with every state onboarded to CELR, many states show persistent data quality issues in their federally-published data that have caused continued disparities with their state-published data.
Throughout the pandemic, both state and federal testing data were treated by health officials and politicians as having precision and comparability they simply did not. State test positivity became the basis of travel ordinances and reopening decisions; federal test positivity was used to inform the federal response; both came with scant acknowledgment of their respective data quality problems, instead creating a din of conflicting information that damaged public trust.
Testing is also the base of the data pipeline for all the other metrics: Many people sought testing for COVID-19 without visiting a clinician, meaning the main point of entry into states’ surveillance systems was a positive COVID-19 test from a lab and not a healthcare provider’s case report. As a result, the weakness of testing pipelines ended up impeding the collection of all other COVID-19 metrics.
Cases
Cases are one of the few COVID-19 metrics for which the federal government has issued clear data standards, but the paths states took toward implementing and adhering to these standards varied greatly. These state-specific paths are important to study because without a standardized way to define a COVID-19 case, it was not always easy to make sound comparisons across states or to produce a national summary.
Testing sits at the heart of these case identification problems. When PCR tests aren’t available—when manufacturing is delayed, when distribution lags, when access to testing sites is limited, and when incentives to seek testing are strained—it becomes crucial to establish another way to build a count. We know that in the first months of the pandemic, probable case identification gaps were especially profound. The CDC’s first probable case definition was difficult for state health departments to work with in practice, since it depended on slow processes like contact tracing. And states were slow to start publicly reporting probable cases. As a result, early probable case counts severely underestimated the number of people likely to have COVID-19.
As states built up their testing programs, and especially as antigen tests began to be deployed as a tool for identifying probable COVID-19 cases, the data grew more and more able to capture a fuller picture of the pandemic. Still, challenges remain. Of the 56 US states and territories we tracked, at least five still report confirmed case numbers only, without disclosing any information about probable cases; a handful more lump probable cases in with their confirmed case counts or don’t make case definitions clear.
What’s more, because the data reporting pipelines needed to send antigen test results to state health officials are brand new, we know that there are still vast numbers of positive antigen test results never making it into state case counts, just like test counts.
Deaths
Like many other countries, the US ended up having two different death counts for COVID-19: the slower but more definitive count released by the CDC’s National Center for Health Statistics, and a faster one compiled from state data.
At the start of the pandemic, the NCHS significantly sped up its process to release provisional death certificate data on deaths due to COVID-19. However, because the provisional death certificate data is charted by date of death, recent weeks display a significant taper effect that can be confusing without good documentation. And NCHS data, because it undergoes a federal review, has generally (but not always) moved slower than state counts.
For a faster picture of mortality, you can turn to state data, which the CDC scraped from state dashboards to assemble its own headline count of COVID-19 deaths. However, at the pandemic’s worst moments, there were still more people dying of COVID-19 than most states’ death reporting infrastructures could handle. Not only did this problem lead to lags in the data, it also caused delays in issuance of death certificates that sometimes blocked relatives of those who died from receiving healthcare coverage or benefits.
The CDC did not issue any guidance about how states should track COVID-19 deaths, leading to a lack of standardization in how states defined the number. Some states counted deaths among individuals who had been identified as having a case of COVID-19, some states counted individuals with death certificates listing COVID-19, and many using a combination of the two. Generally, states seemed to choose the method that was fastest for them within the constraints of their case surveillance and death infrastructures. And though it’s a common refrain that “deaths among cases” might overcount COVID-19 deaths, states using each method ended up undercounting NCHS death certificate data by approximately the same amount.
Though these two methods ended up counting deaths at roughly the same speed and comprehensiveness, the federal government did not properly explain that states used different processes to count COVID-19 deaths; instead, at different times, the CDC seemed conflicted about the definition of the count, saying in its data FAQ that state numbers represent deaths among cases identified according to the CSTE definition, and in a statement to us that the counts represent death certificate data. And because states did not receive any guidance from CDC on how to report deaths, not all states initially chose their counting methods with an eye toward speed. As a result, some had to switch to faster methods for counting deaths midway through the pandemic, causing significant confusion and sometimes distrust when numbers abruptly changed.
Hospitalizations
Like other COVID-19 metrics, definitional differences hampered hospitalization data reporting across the country. There was little standardization in how states reported current or cumulative patients, patients with confirmed or suspected cases, and pediatric cases. Many states didn’t readily define metrics on their websites, and many hospitals simply weren’t providing data.
In July 2020, confusion grew when the Trump administration issued a sweeping order that fundamentally changed how COVID-19 hospitalization data was being compiled. In addition to reporting information to state health departments, hospitals across the country were suddenly directed to report COVID-19 numbers to the US Department of Health and Human Services, which oversees the CDC, instead of reporting to the CDC directly.
At first, the switch was challenged, to say the least. (We wrote about the initial effects on the data here.) But as we watched hospitalization data closely over the second half of 2020, studying it to see how it tracked with numbers we were gathering from states themselves, we saw that the new protocol in effect had patched the places where crucial data had been missing. In fact, current hospitalization data grew to be so reliably well-reported—and federal data tracked with ours so closely—that the metric became a kind of lodestar in our understanding of the trajectory of the pandemic.
Finally, in November 2020, we decided to remove the “cumulative hospitalization” metric from our website. We knew that data from the early months of the pandemic was drastically incomplete, and we watched as many states had cumulative totals that sat stagnant for weeks, while their current hospitalization numbers fluctuated. Additionally, 20 states never reported cumulative hospitalizations, making the national sum a large undercount. Ultimately, we decided that reporting the cumulative number of COVID-19 patients hospitalized was helpful in theory but less so in practice, and we tried to guide our data users toward more valuable metrics, like current hospitalization and new hospital admissions numbers instead.
Recoveries
Our last of the five major metrics is one that sounds intrinsically hopeful and good, but in reality, it’s just as complicated as the others: recoveries.
Unfortunately, the recoveries metric shares many of the same challenges seen across COVID-19 data—it’s poorly defined, unstandardized, not reported in every state, and difficult to fully capture when case counts grow to scales that overwhelm state health departments.
What’s more, an additional layer of complexity looms over the recoveries metric, presenting a kind of philosophical dilemma. Scientists are still learning about the long-term health effects of COVID-19, even among asymptomatic cases. Declaring an individual “recovered” simply because they avoided death can be misleading and insensitive.
For all these reasons, The COVID Tracking Project stopped reporting a national summary of recovery figures in November 2020 and decided to remove state-level recovery figures from our website in January 2021. Instead of providing figures for recoveries, we began to track and display hospital discharges for the eight states providing it, which had a clearer, more standardized meaning across states. As we wrote about state recovered metrics, our recommendation is that state health officials carefully consider how they discuss and quantify this information, choosing metrics like “Released from Isolation” or “Inactive Cases” over labels that imply full recovery.
What we learned, and what we hope happens next
Over the past two months, a small crew at The COVID Tracking Project has been working to document our year of data collection, reflecting on how best to organize the history of our project so that journalists, policymakers, advocates, and the public might continue to find relevance in our work.
As we pored over our research on state reporting, we congealed our findings into a set of common reporting problems that made COVID-19 data especially difficult to aggregate on a national level. States tended to differ on how they defined data, what data they made available, and how they presented what data they did publish, making it difficult to compare data across states. All of those themes come through in the reporting arcs of these five COVID-19 metrics.
Some of these problems could have been avoided with clearer reporting guidance from the federal government; others were inevitable, given the constraints of the United States’ underfunded public health infrastructure. But all of them tended to be poorly documented, meaning it took a great deal of excavation to uncover the sources of these problems—or even that these problems existed in the first place.
These data challenges may have been readily apparent or even expected to those familiar with the contours of public health informatics. But pandemics affect us all, and the infrastructure that responds to them is meant to protect us all, so we all deserve to understand how capable the infrastructure is. Frankly, we need to understand its limitations to navigate through a pandemic.
Above and beyond any individual reporting practice, we believe it was the lack of explanations that led to misuse of the data and wounded public trust. We tried our best to provide explanations where possible, and we saw transformation when we were able to get through. Data users who were frustrated or even doubtful came to trust the numbers. Journalists reported more accurately. Hospitals could better anticipate surges.
If we could make just one change to how state and federal COVID-19 data was reported, it would be to make an open acknowledgement of the limitations of public health data infrastructure whenever the data is presented. And if we could make one plea for what comes next, it’s that these systems receive the continued investment they deserve so that they don’t get in the way of our view of critical public health data.
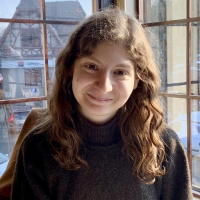
Kara Schechtman is Data Quality Co-Lead for The COVID Tracking Project.

Sara Simon works on The COVID Tracking Project’s data quality team and is also a contributing writer. She most recently worked as an investigative data reporter at Spotlight PA and software engineer at The New York Times.
More “Testing Data” posts
How Probable Cases Changed Through the COVID-19 Pandemic
When analyzing COVID-19 data, confirmed case counts are obvious to study. But don’t overlook probable cases—and the varying, evolving ways that states have defined them.
20,000 Hours of Data Entry: Why We Didn’t Automate Our Data Collection
Looking back on a year of collecting COVID-19 data, here’s a summary of the tools we automated to make our data entry smoother and why we ultimately relied on manual data collection.
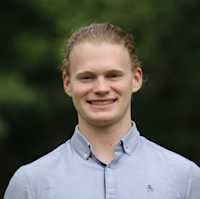
Releasing Our State COVID-19 Data Log
From July 2020 to March 2021, The COVID Tracking Project compiled a detailed set of structured COVID-19 data notes, both on changes states made to the data and changes we made to the data we captured from states. Today, we’re releasing those notes.
